When Hype Cycles Meet S-Curves: The Roll-Out Conundrum of Generative AI
When Hype Cycles Meet S-Curves: The Roll-Out Conundrum of Generative AI
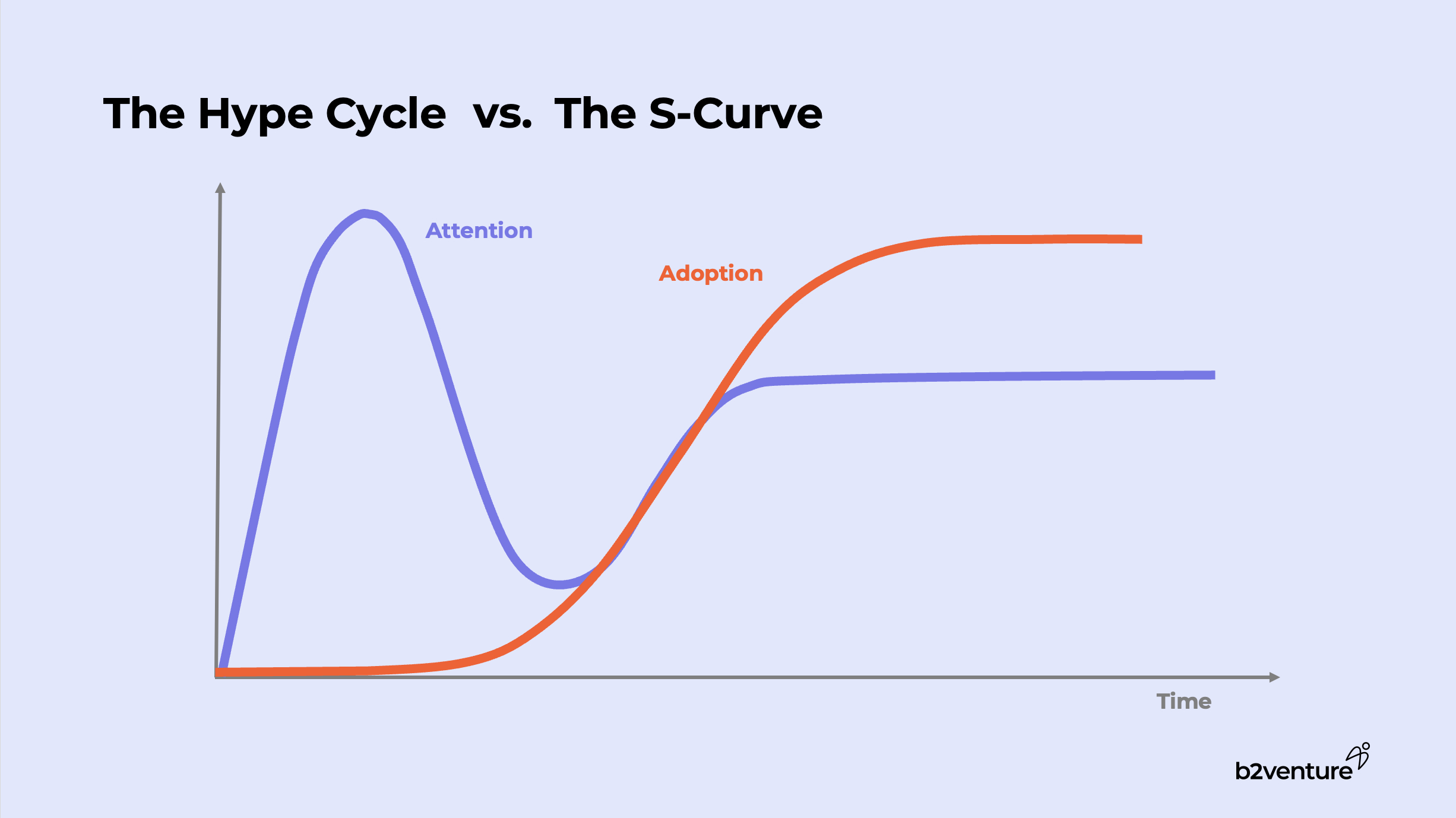
Hype cycles have long been part of how new technologies unfold, and there’s no doubt that the generative AI hype cycle is in full swing. Barely a day passes without some small or large technical break-throughs in fields such as AI chips, large language models or video generation. One blockbuster funding announcement for a promising AI startup is followed by another. And the numbers have become eye-wateringly large, with Open AI’s Sam Altman allegedly now looking to raise trillions of dollars to build out AI infrastructure.
Yet all this exuberance stands in sharp contrast with the commercial reality on the ground. Some early AI unicorns, such as Inflection, already had to abandon their original mission, while others seem to be in serious trouble. Even the big cloud hyperscalers such as Google and AWS seem to be tempering expectations for rapid revenue growth in generative AI. Critical voices about the usefulness and cost effectiveness of generative AI applications are also increasing.
Apps for individuals seem to be doing fine — one example is our portfolio company, TextCortex, which offers an advanced, highly customizable AI co-pilot that is primarily used by individual users in various professions. It was recently ranked as one of the top 20 fastest growing software products by review site G2, alongside some other startups that offer generative AI capabilities mostly to individual users. Individuals are quick to adopt new tools that help them be more productive, and they are willing to pay for them. Often that’s the first step towards broader adoption, yet with some important caveats I will explain in more detail below.
Despite the excitement, enterprises have been slow to meaningfully adopt AI
On the surface, the excitement around generative AI has set off a wildfire of interest in the corporate world too, yet this has yielded very little when you drill down. Most corporations seem to be experimenting heavily, often with small teams executing proof-of-concept projects. Bigger enterprises bring in scores of consultants to build first-generation applications. System integrator Accenture, for example, just announced $600m in revenue from AI projects in just one quarter.
But whenever we talk with people in the enterprise segment in detail about AI projects, they all tell us a similar story: very few of these proofs-of-concept ever make it into production. When you look at what most enterprises are really using on a day-to-day basis for mission-critical tasks or workflows, it’s often just a handful of models in very traditional fields such as risk modeling or predictive analytics. Fancy co-pilots enabling dramatic productivity gains are nowhere to be seen.
So, what’s going on here? Why are companies not deploying AI much more quickly, if it’s obviously useful and getting so much attention from front-line workers all the way to the C-suite?
The hype cycle operates on a different time scale than real-world adoption
The divergence between the hype cycle and real-world adoption across industries and markets is nothing new. We experience this with every new technology, even if the levels of hype differ.
A good way to explain this is by contrasting a typical hype cycle with the reality of technology deployment which follows an S-curve.
The hype cycle was coined by market research firm Gartner and explains the wild swings in market mood whenever a new technology emerges. Initiated by a technology trigger (“the cool new thing”), expectations rise very quickly to a peak of inflated expectations. That’s presumably where we are now with generative AI. All the breathless articles about how this technology is going to change everything, plus the vast amounts of money that are being invested, are a sure sign of a peak. And the challenges outlined above are an indicator that generative AI could be slowly tipping into a decline of expectations.
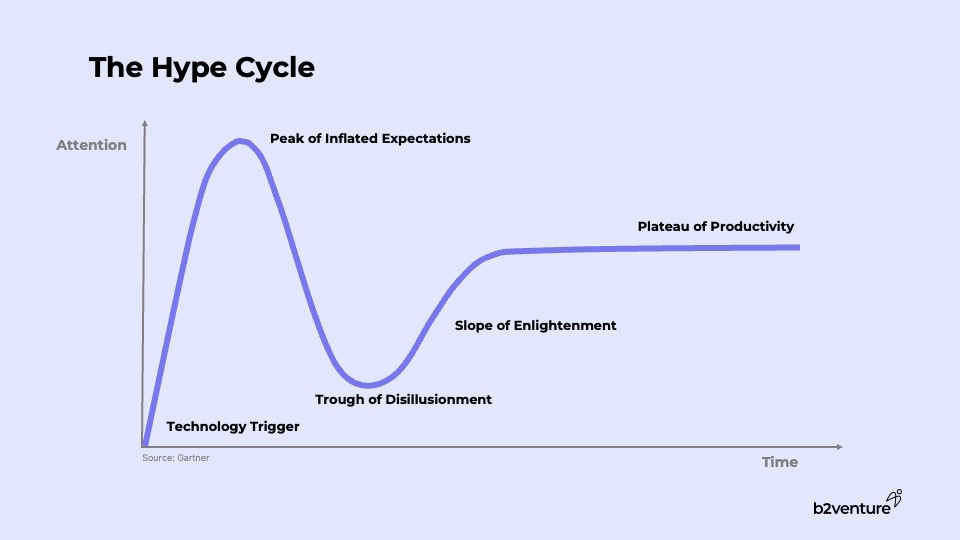
Typically the mood collapses quite quickly when people figure out that the new technology will need more time than anticipated to live up to expectations.The peak is followed by deep disillusionment, only to then slowly recover to a plateau of productivity where expectations meet reality. Older AI technologies such as predictive analytics, computer vision or speech recognition are now mostly at this steady state, but generative AI will go through the wild gyrations of perception captured by the hype cycle model.
The actual deployment of technology in the real world follows a different pattern: that of an S-curve. It is probably the one of the most frequent patterns in innovation and yet very hard to predict at the same time. In terms of deployment of generative AI, we are without a doubt still at the initial plateau of the S-curve. A few innovators are using these tools daily, some others are playing with them, but we are far away from broader adoption.
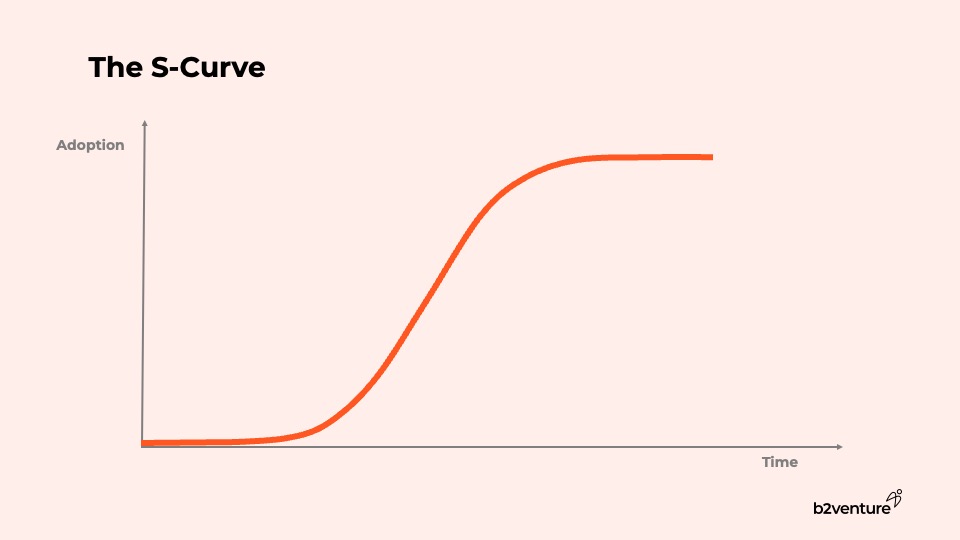
The beauty of the S-curve, however, is that once a technology reaches a tipping point, things develop very quickly. For example, the introduction of the iPhone in 2007 was likely the tipping point for smartphones, a category that had been around for a decade. Within another decade, the market expanded dramatically from its niche as a tool for business execs to mass market phenomenon. By 2018, 3 billion people were using a smartphone, and today there are 5.5 billion users. In best S-curve fashion, smartphone sales have already flattened out since the market became saturated.
What’s holding back adoption of generative AI today?
There is little doubt that generative AI will unfold according to this familiar pattern - with broader adoption perhaps accelerating even more quickly than past waves of innovation - yet what’s holding this adoption curve back right now?
We’ve developed a few hypotheses as to why:
- It’s still hard for companies to understand where generative AI could be most effective for their business. If history is any guide, it typically takes more than a decade to figure out what the best use cases for a new technology are. Some ideas and approaches that appear promising now will turn out to be largely useless, while some of the best use cases probably have not been invented yet.
- Generative AI capabilities are developing rapidly, and there is a lot of uncertainty about use cases that can already be properly supported versus those that are still purely experimental. Expensive IT projects need a high degree of technological certainty, and that’s not what the current generation of generative AI can offer yet. Proof-of-concept projects will continue, but for large scale deployments in production, the technology will have to stabilize further.
- AI talent has always been hard to hire, but now it’s more difficult than ever. With many well-financed startups snatching up the best AI engineers, it’s hard for corporations to build up a strong AI team internally. Even large consultancies are struggling to find enough people with the right expertise.
- End users have to learn how to use generative AI tools as well. Products such as AI co-pilots depend very much on the individual user’s understanding of a new tool’s potential and their willingness to adapt and invest the time to understand how to best use them. This might turn out to be a slow process since many people are reluctant to change the way they work, if the product is complex and there’s uncertainty about the ultimate gains.
- Given all of these restrictions, making an ROI case for generative AI in any business context is incredibly hard. The prices of input factors keep changing, and the effectiveness of the output is murky.
How long is it going to take to reach convergence?
While these are very serious challenges, we’ve seen this story before in previous technology waves as the hype cycle and s-curve converge over time.
For example, the adoption of cloud computing by large corporations was very slow initially. Just about 5% of IT workloads had been migrated to the cloud in 2015 — nine years after the initial release of AWS. According to most estimates, today about 60% of enterprise IT workloads are cloud-based, with cloud services continuing to grow very rapidly (and profitably).
Ironically, the tipping point of the S-curve often happens when the hype curve is at its lowest point. That’s when the noise has dissipated and dedicated practitioners are working out exactly what a new technology can really do that’s unique and unlocks new efficiencies.
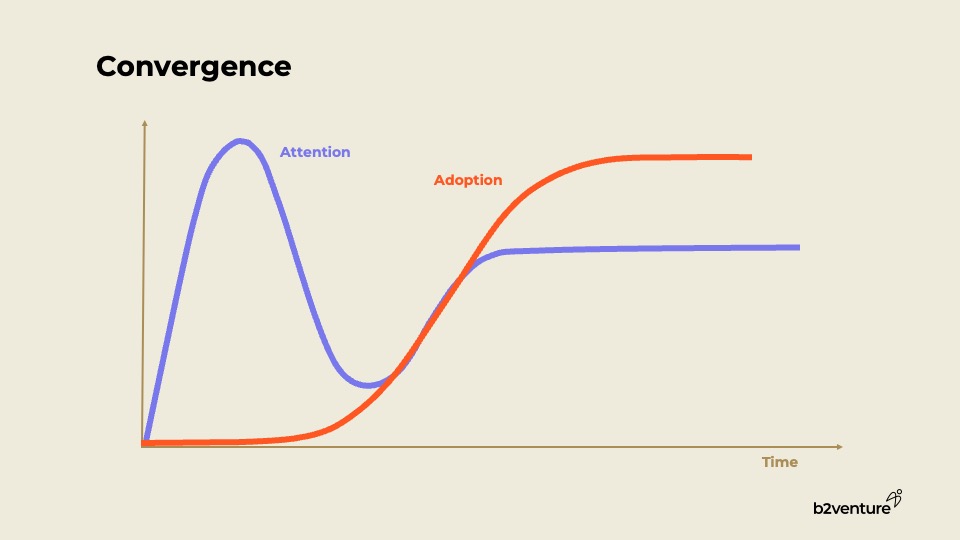
How long will this process take for generative AI?
It’s hard to tell because there are two opposing forces at work:
- The speed of innovation in generative AI is much higher than in previous technology waves, suggesting that the technology could mature quickly and that more use cases with dramatic ROI might become possible soon.
- On the other hand, the technology is much more abstract, harder to understand and more capital intensive. Smartphones or cloud computing were relatively straightforward. Generative AI is more like the Internet: A very broad technology with countless applications. The market may need time to mature with enough people that have the skills and ability to figure out what the best use cases will be.
How should AI startups address this market uncertainty on the road to convergence?
First of all, startups shouldn’t put too much hope into traditional methods of customer and market discovery. This is not SaaS, where finding product-market fit is often all about discovering an unmet customer need and then digitizing it with a well-known toolset and business model.
We are truly in unchartered territory here. Generative AI is a transformative technology, not an incremental innovation. No amount of customer interviews will give you much more clarity in this market because your potential customers are just figuring things out themselves.
If you study the history of technology, you will discover three paths to success as a startup in a new tech wave:
- Tech pioneers: Be among the very first that use a new technology and help define an entirely new category. Apple and Microsoft are examples. When they started, the market for personal computers simply didn’t exist. Salesforce was one of the very first SaaS companies and ended up dominating the CRM industry (and SaaS with it).
- Fast followers that get it right: Enter an existing (but still young) category and sell a much better product. Google and Facebook are classic examples. Google had about 20 direct competitors when it released its first search engine, but the product was just clearly better.
- Sector disruptors: Use the technology to build a disruptive service in a slow incumbent industry outside of the tech world. Amazon (web-based “mail order” commerce) and Uber (smartphone-based taxi service) are iconic cases for this strategy.
There is little doubt that we are going to see similar patterns in the generative AI wave. Some of the early pioneers are likely going to be dominant companies, but as usual it’s hard to predict which ones. It is also highly likely that we haven’t seen the generative AI equivalents of Google or Facebook yet. They are going to be founded in the next few years.
And finally, disruptive business models that are enabled by generative AI might turn out to be the most exciting field. There is already a lot of talk about companies that sell an AI-enabled service work product, not the underlying software. For example, what would an AI-first law firm look like? Could it sell legal services at a fraction of the price, at a higher quality and at a dramatically higher speed than a traditional law firm?
These three patterns address the adoption gap described above in different ways. Path 1 (Microsoft) accepts that adoption is going to take time, but the potential outcome could be huge. Path 2 (Google) taps into sectors that already have demonstrated strong technology adoption and uses a clear product advantage to capture the market. Path 3 (Uber) shortcuts the adoption curve by not selling technology, but a more efficient product in a sector with well-known demand.
Bold moves needed for the interesting times ahead
The bottom line is that founders in generative AI need to be more creative and much bolder than those who played in traditional SaaS over the last decade. The traditional software industry has a well-defined playbook with well-understood economics. The business model of a generative AI company, on the other hand, is more complex with many more dimensions. Basic factors such as typical gross margins, customers’ willingness to pay or expected churn rates are largely unknown at this point.
Figuring this out will require a combination of rapid iteration, bold leaps, and plenty of patience. That’s a challenge - not just for founders, but also for employees and investors.
Some things that generative AI founders will need to do in order to survive the adoption gap:
- Be bold and define what the product should be in your opinion, instead of what customers are asking for now. That’s not the same as blind technology push, but a very iterative process of identifying technical opportunities that can take a product into a direction that customers will probably want without them really knowing it now. The classic example is the iPhone: Apple was bold enough to defy the conventional wisdom of the time (users want physical keyboards on their phones) because there was a technical opportunity (precise multi-touch touchscreens).
- Find early lighthouse customers who are ahead of their time. The good old “crossing the chasm” framework deserves to be revisited. In any immature market, you will find a few innovators who understand what a new technology might be useful for and are willing to pay for it, even under uncertainty. You can learn a lot from these kinds of customers, and they will give credibility to your value proposition.
- Focus on a main direction for your product but remain open for alternatives with a few small “side bets”. In immature markets, it’s not unusual to take a path that looks plausible, but ultimately leads to nowhere. Smart founders hedge their bets without losing focus. If you need to pivot because the original thesis doesn’t work, it’s good to have alternatives.
- Think about an ROI story early and consistently. Early adopter customers will want to understand the ROI of your product, and they will need guidance. Focusing on a tangible ROI will also guide your product decisions into a direction that will speed up commercialization of your technology.
These are indeed interesting times in many ways for entrepreneurs in the generative AI field. It may feel like the Wild West right now, but it’s moments like these when the most impressive companies are built.
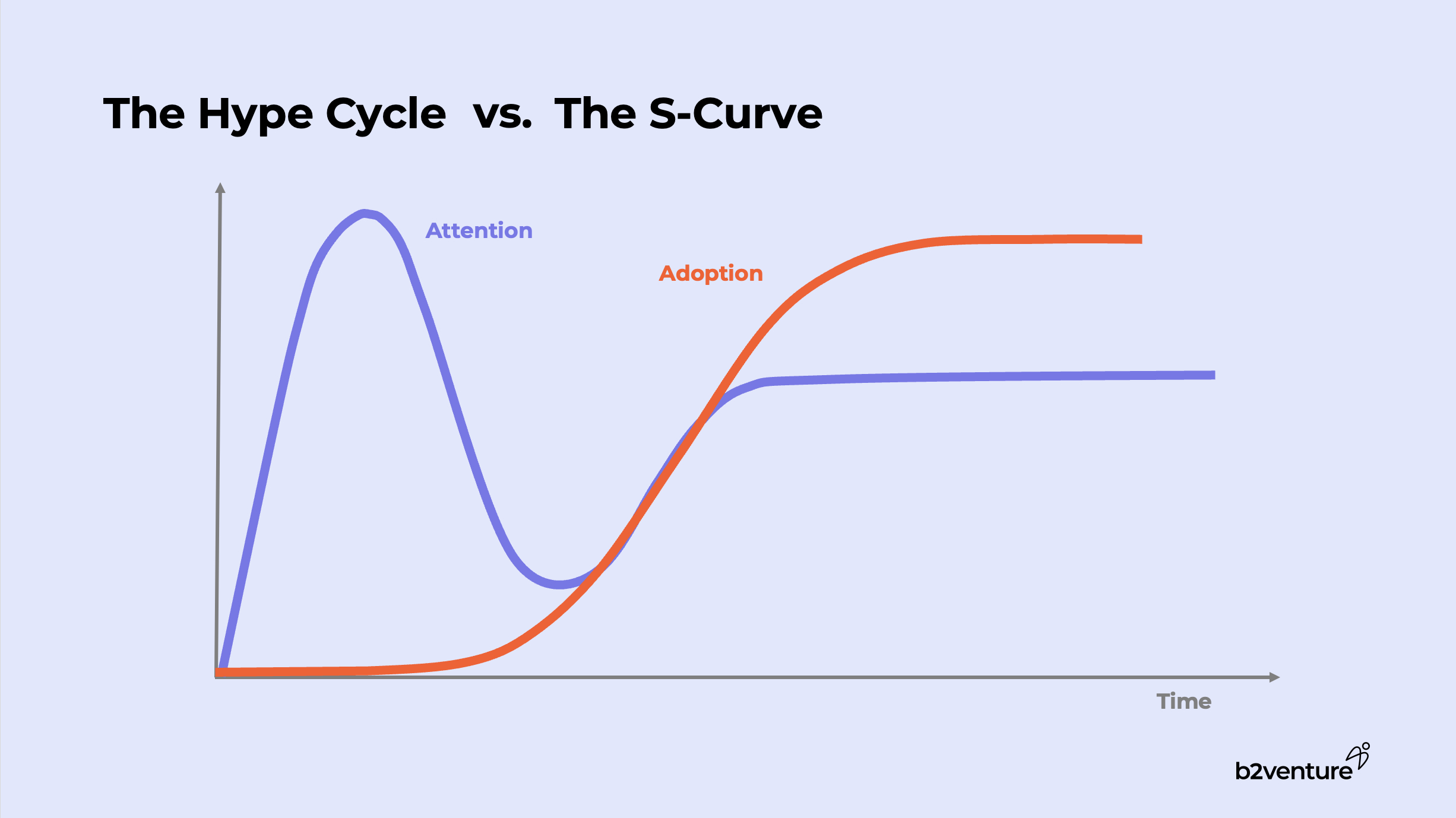
Hype cycles have long been part of how new technologies unfold, and there’s no doubt that the generative AI hype cycle is in full swing. Barely a day passes without some small or large technical break-throughs in fields such as AI chips, large language models or video generation. One blockbuster funding announcement for a promising AI startup is followed by another. And the numbers have become eye-wateringly large, with Open AI’s Sam Altman allegedly now looking to raise trillions of dollars to build out AI infrastructure.
Yet all this exuberance stands in sharp contrast with the commercial reality on the ground. Some early AI unicorns, such as Inflection, already had to abandon their original mission, while others seem to be in serious trouble. Even the big cloud hyperscalers such as Google and AWS seem to be tempering expectations for rapid revenue growth in generative AI. Critical voices about the usefulness and cost effectiveness of generative AI applications are also increasing.
Apps for individuals seem to be doing fine — one example is our portfolio company, TextCortex, which offers an advanced, highly customizable AI co-pilot that is primarily used by individual users in various professions. It was recently ranked as one of the top 20 fastest growing software products by review site G2, alongside some other startups that offer generative AI capabilities mostly to individual users. Individuals are quick to adopt new tools that help them be more productive, and they are willing to pay for them. Often that’s the first step towards broader adoption, yet with some important caveats I will explain in more detail below.
Despite the excitement, enterprises have been slow to meaningfully adopt AI
On the surface, the excitement around generative AI has set off a wildfire of interest in the corporate world too, yet this has yielded very little when you drill down. Most corporations seem to be experimenting heavily, often with small teams executing proof-of-concept projects. Bigger enterprises bring in scores of consultants to build first-generation applications. System integrator Accenture, for example, just announced $600m in revenue from AI projects in just one quarter.
But whenever we talk with people in the enterprise segment in detail about AI projects, they all tell us a similar story: very few of these proofs-of-concept ever make it into production. When you look at what most enterprises are really using on a day-to-day basis for mission-critical tasks or workflows, it’s often just a handful of models in very traditional fields such as risk modeling or predictive analytics. Fancy co-pilots enabling dramatic productivity gains are nowhere to be seen.
So, what’s going on here? Why are companies not deploying AI much more quickly, if it’s obviously useful and getting so much attention from front-line workers all the way to the C-suite?
The hype cycle operates on a different time scale than real-world adoption
The divergence between the hype cycle and real-world adoption across industries and markets is nothing new. We experience this with every new technology, even if the levels of hype differ.
A good way to explain this is by contrasting a typical hype cycle with the reality of technology deployment which follows an S-curve.
The hype cycle was coined by market research firm Gartner and explains the wild swings in market mood whenever a new technology emerges. Initiated by a technology trigger (“the cool new thing”), expectations rise very quickly to a peak of inflated expectations. That’s presumably where we are now with generative AI. All the breathless articles about how this technology is going to change everything, plus the vast amounts of money that are being invested, are a sure sign of a peak. And the challenges outlined above are an indicator that generative AI could be slowly tipping into a decline of expectations.
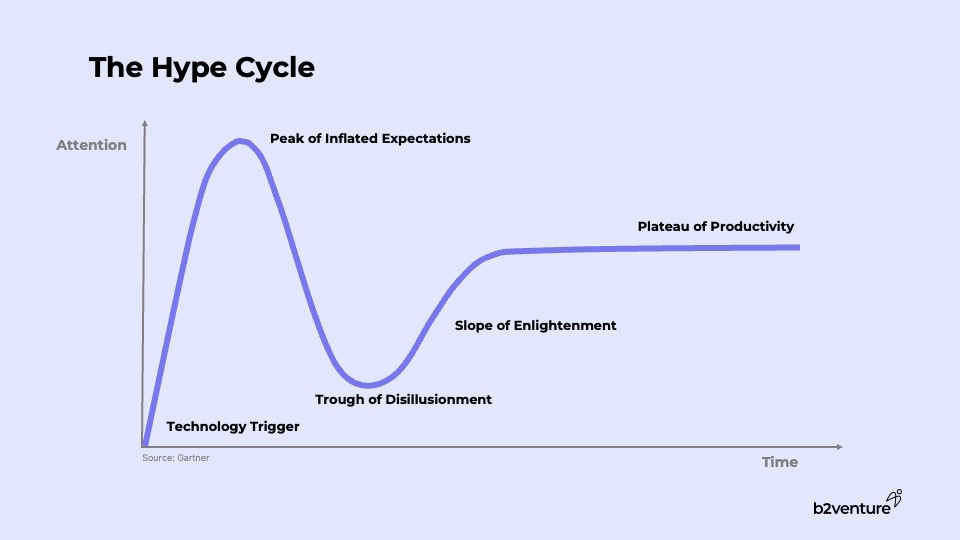
Typically the mood collapses quite quickly when people figure out that the new technology will need more time than anticipated to live up to expectations.The peak is followed by deep disillusionment, only to then slowly recover to a plateau of productivity where expectations meet reality. Older AI technologies such as predictive analytics, computer vision or speech recognition are now mostly at this steady state, but generative AI will go through the wild gyrations of perception captured by the hype cycle model.
The actual deployment of technology in the real world follows a different pattern: that of an S-curve. It is probably the one of the most frequent patterns in innovation and yet very hard to predict at the same time. In terms of deployment of generative AI, we are without a doubt still at the initial plateau of the S-curve. A few innovators are using these tools daily, some others are playing with them, but we are far away from broader adoption.
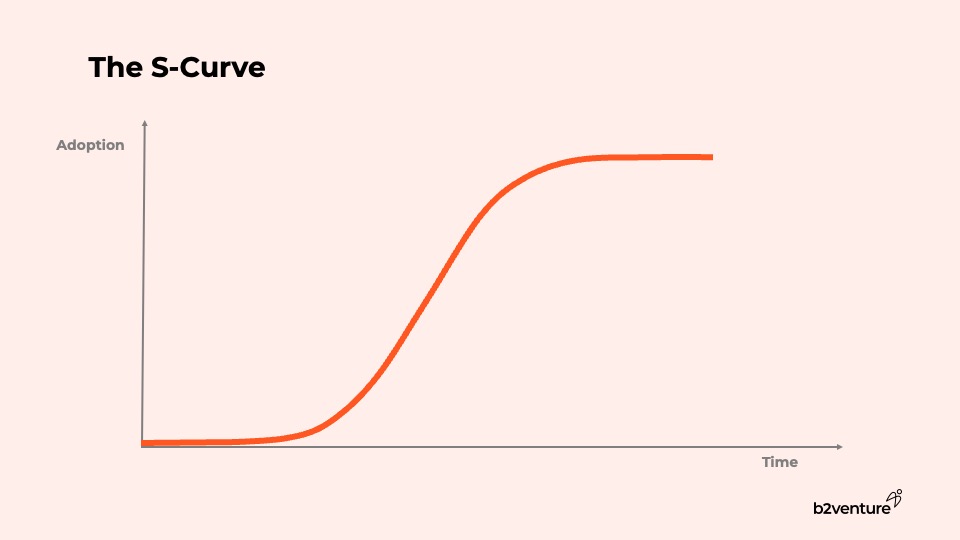
The beauty of the S-curve, however, is that once a technology reaches a tipping point, things develop very quickly. For example, the introduction of the iPhone in 2007 was likely the tipping point for smartphones, a category that had been around for a decade. Within another decade, the market expanded dramatically from its niche as a tool for business execs to mass market phenomenon. By 2018, 3 billion people were using a smartphone, and today there are 5.5 billion users. In best S-curve fashion, smartphone sales have already flattened out since the market became saturated.
What’s holding back adoption of generative AI today?
There is little doubt that generative AI will unfold according to this familiar pattern - with broader adoption perhaps accelerating even more quickly than past waves of innovation - yet what’s holding this adoption curve back right now?
We’ve developed a few hypotheses as to why:
- It’s still hard for companies to understand where generative AI could be most effective for their business. If history is any guide, it typically takes more than a decade to figure out what the best use cases for a new technology are. Some ideas and approaches that appear promising now will turn out to be largely useless, while some of the best use cases probably have not been invented yet.
- Generative AI capabilities are developing rapidly, and there is a lot of uncertainty about use cases that can already be properly supported versus those that are still purely experimental. Expensive IT projects need a high degree of technological certainty, and that’s not what the current generation of generative AI can offer yet. Proof-of-concept projects will continue, but for large scale deployments in production, the technology will have to stabilize further.
- AI talent has always been hard to hire, but now it’s more difficult than ever. With many well-financed startups snatching up the best AI engineers, it’s hard for corporations to build up a strong AI team internally. Even large consultancies are struggling to find enough people with the right expertise.
- End users have to learn how to use generative AI tools as well. Products such as AI co-pilots depend very much on the individual user’s understanding of a new tool’s potential and their willingness to adapt and invest the time to understand how to best use them. This might turn out to be a slow process since many people are reluctant to change the way they work, if the product is complex and there’s uncertainty about the ultimate gains.
- Given all of these restrictions, making an ROI case for generative AI in any business context is incredibly hard. The prices of input factors keep changing, and the effectiveness of the output is murky.
How long is it going to take to reach convergence?
While these are very serious challenges, we’ve seen this story before in previous technology waves as the hype cycle and s-curve converge over time.
For example, the adoption of cloud computing by large corporations was very slow initially. Just about 5% of IT workloads had been migrated to the cloud in 2015 — nine years after the initial release of AWS. According to most estimates, today about 60% of enterprise IT workloads are cloud-based, with cloud services continuing to grow very rapidly (and profitably).
Ironically, the tipping point of the S-curve often happens when the hype curve is at its lowest point. That’s when the noise has dissipated and dedicated practitioners are working out exactly what a new technology can really do that’s unique and unlocks new efficiencies.
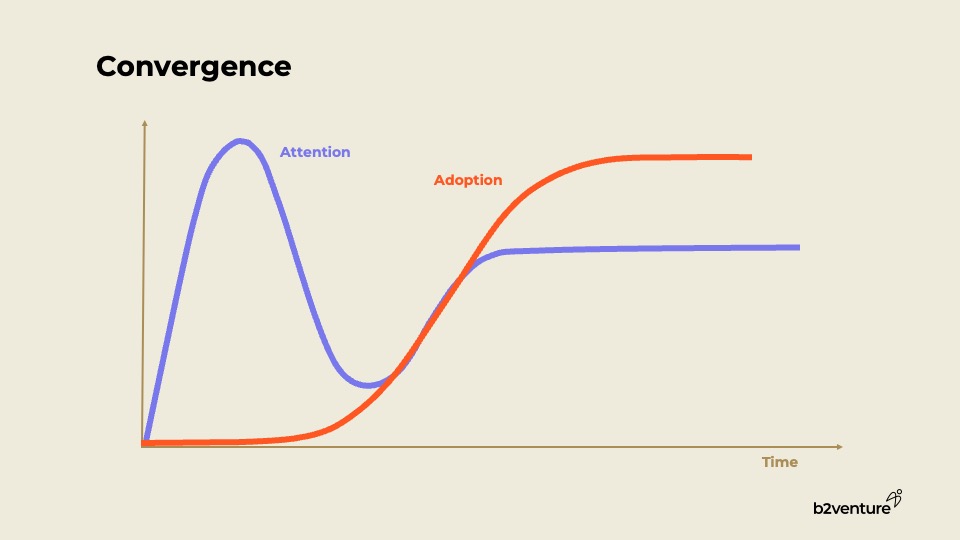
How long will this process take for generative AI?
It’s hard to tell because there are two opposing forces at work:
- The speed of innovation in generative AI is much higher than in previous technology waves, suggesting that the technology could mature quickly and that more use cases with dramatic ROI might become possible soon.
- On the other hand, the technology is much more abstract, harder to understand and more capital intensive. Smartphones or cloud computing were relatively straightforward. Generative AI is more like the Internet: A very broad technology with countless applications. The market may need time to mature with enough people that have the skills and ability to figure out what the best use cases will be.
How should AI startups address this market uncertainty on the road to convergence?
First of all, startups shouldn’t put too much hope into traditional methods of customer and market discovery. This is not SaaS, where finding product-market fit is often all about discovering an unmet customer need and then digitizing it with a well-known toolset and business model.
We are truly in unchartered territory here. Generative AI is a transformative technology, not an incremental innovation. No amount of customer interviews will give you much more clarity in this market because your potential customers are just figuring things out themselves.
If you study the history of technology, you will discover three paths to success as a startup in a new tech wave:
- Tech pioneers: Be among the very first that use a new technology and help define an entirely new category. Apple and Microsoft are examples. When they started, the market for personal computers simply didn’t exist. Salesforce was one of the very first SaaS companies and ended up dominating the CRM industry (and SaaS with it).
- Fast followers that get it right: Enter an existing (but still young) category and sell a much better product. Google and Facebook are classic examples. Google had about 20 direct competitors when it released its first search engine, but the product was just clearly better.
- Sector disruptors: Use the technology to build a disruptive service in a slow incumbent industry outside of the tech world. Amazon (web-based “mail order” commerce) and Uber (smartphone-based taxi service) are iconic cases for this strategy.
There is little doubt that we are going to see similar patterns in the generative AI wave. Some of the early pioneers are likely going to be dominant companies, but as usual it’s hard to predict which ones. It is also highly likely that we haven’t seen the generative AI equivalents of Google or Facebook yet. They are going to be founded in the next few years.
And finally, disruptive business models that are enabled by generative AI might turn out to be the most exciting field. There is already a lot of talk about companies that sell an AI-enabled service work product, not the underlying software. For example, what would an AI-first law firm look like? Could it sell legal services at a fraction of the price, at a higher quality and at a dramatically higher speed than a traditional law firm?
These three patterns address the adoption gap described above in different ways. Path 1 (Microsoft) accepts that adoption is going to take time, but the potential outcome could be huge. Path 2 (Google) taps into sectors that already have demonstrated strong technology adoption and uses a clear product advantage to capture the market. Path 3 (Uber) shortcuts the adoption curve by not selling technology, but a more efficient product in a sector with well-known demand.
Bold moves needed for the interesting times ahead
The bottom line is that founders in generative AI need to be more creative and much bolder than those who played in traditional SaaS over the last decade. The traditional software industry has a well-defined playbook with well-understood economics. The business model of a generative AI company, on the other hand, is more complex with many more dimensions. Basic factors such as typical gross margins, customers’ willingness to pay or expected churn rates are largely unknown at this point.
Figuring this out will require a combination of rapid iteration, bold leaps, and plenty of patience. That’s a challenge - not just for founders, but also for employees and investors.
Some things that generative AI founders will need to do in order to survive the adoption gap:
- Be bold and define what the product should be in your opinion, instead of what customers are asking for now. That’s not the same as blind technology push, but a very iterative process of identifying technical opportunities that can take a product into a direction that customers will probably want without them really knowing it now. The classic example is the iPhone: Apple was bold enough to defy the conventional wisdom of the time (users want physical keyboards on their phones) because there was a technical opportunity (precise multi-touch touchscreens).
- Find early lighthouse customers who are ahead of their time. The good old “crossing the chasm” framework deserves to be revisited. In any immature market, you will find a few innovators who understand what a new technology might be useful for and are willing to pay for it, even under uncertainty. You can learn a lot from these kinds of customers, and they will give credibility to your value proposition.
- Focus on a main direction for your product but remain open for alternatives with a few small “side bets”. In immature markets, it’s not unusual to take a path that looks plausible, but ultimately leads to nowhere. Smart founders hedge their bets without losing focus. If you need to pivot because the original thesis doesn’t work, it’s good to have alternatives.
- Think about an ROI story early and consistently. Early adopter customers will want to understand the ROI of your product, and they will need guidance. Focusing on a tangible ROI will also guide your product decisions into a direction that will speed up commercialization of your technology.
These are indeed interesting times in many ways for entrepreneurs in the generative AI field. It may feel like the Wild West right now, but it’s moments like these when the most impressive companies are built.
The Author
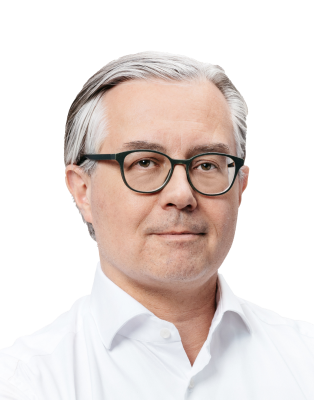
Andreas Goeldi
Partner
Andreas Goeldi is Partner and has been part of the b2venture Fund Team since 2019. He is an avid technologist, serial entrepreneur, and investor with over 25 years’ experience.
Team