Generative AI: An Investor's Perspective
Generative AI: An Investor's Perspective
Back in 2017, Nvidia CEO Jensen Huang predicted a "Cambrian explosion of autonomous machines'', and the growth of specialized hardware, software, and machine learning models over the last several years have indeed led to an abundance of tools and technologies we associate with "artificial intelligence".
While many in the tech world have been interested in AI for a long time, the immense popularity of ChatGPT has kicked off a tremendous new wave of investment in AI startups and enormous interest in generative AI and large language models (LLMs).
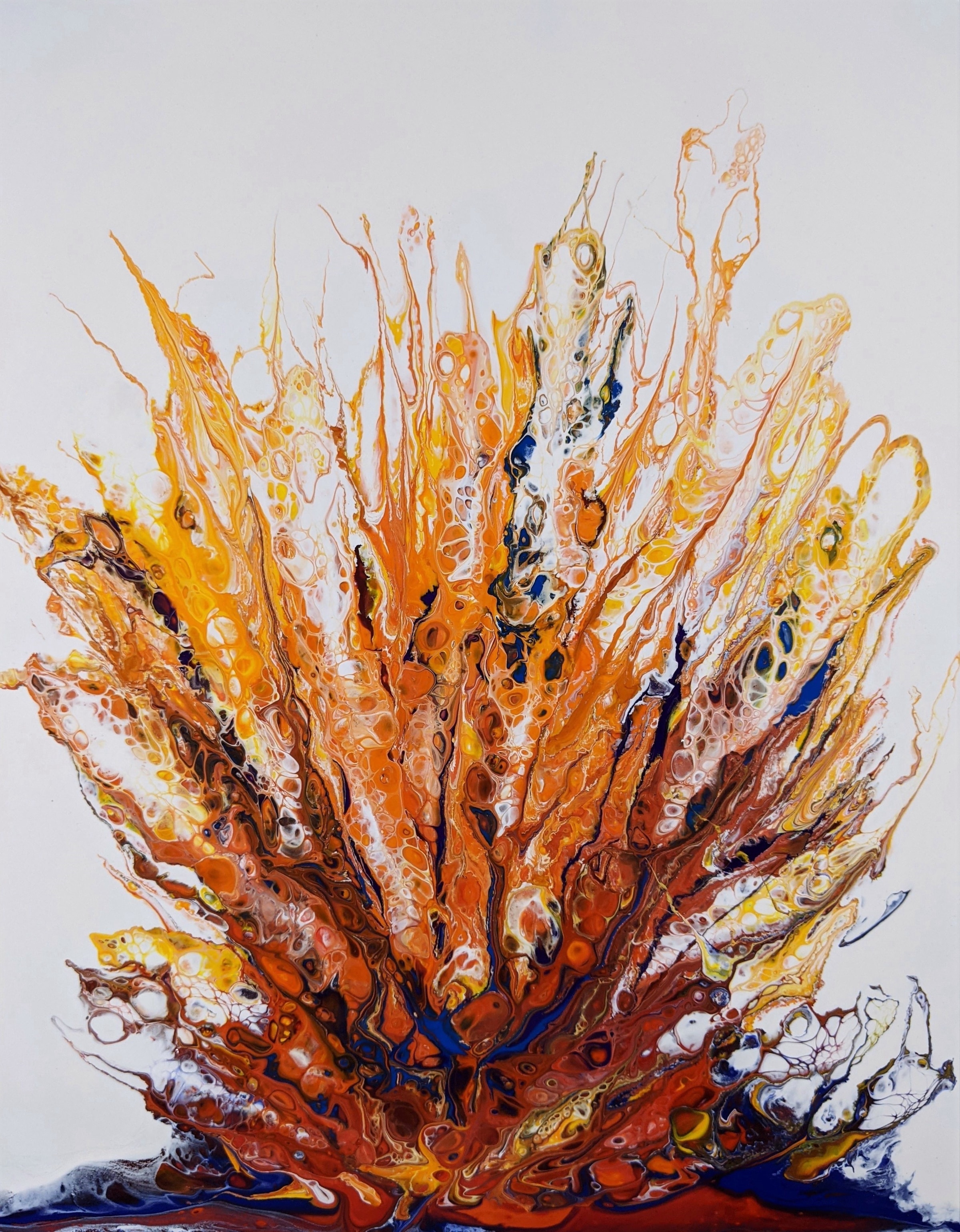
As one of the most active AI investors in Europe, b2venture has been investing in AI-related companies for a long time when we made our first investment in the translation engine DeepL – from today’s perspective, one of the very first generative AI companies - and since then a host of others, including LatticeFlow, Chattermill, Textcortex, Calvin Risk, Neptune AI, and Decentriq.
Over the coming months, we will be publishing our thoughts on how we see the market on AI developing (including the occasional experiment with new and emergent AI tools, such as Andreas Goeldi’s post about AI and venture capital.).
Our Approach
Historically, our approach to AI has been based on several dimensions:
- Long-term momentum: we are interested in sectors within AI that are fundamentally enabling technologies and will therefore see sustained growth over an extended period.
- Competitive intensity and structure: we evaluate the nature of competition within different subsectors of AI and try to understand how the market is structured, considering factors such as the number of players, market share, and barriers to entry. A key consideration is the role of incumbents that might adopt new AI technologies, as currently seen in the cases of Microsoft and Adobe.
- Business model opportunities: We consider various business models, such as open-source (where code is freely available and needs to be monetized indirectly) versus closed source (proprietary code that is sold as a product), analyzing the advantages and disadvantages of these different approaches.
- Defensibility: We assess the level of technical difficulty and the barriers to entry that could create a competitive advantage or "moat" for certain subsectors. Higher technical difficulty often offers more defensibilty and better long-term prospects, but comes at the price of requiring more capital and often longer adoption curves.
- Avoiding short-term trends (JOMO - "Joy of Missing Out"): we deliberately avoid short-term trends that may be popular but have a higher likelihood of failure in the long run. One example is "no-code" tools and platforms that allow people to create applications without coding, as these have demonstrable shortcomings and rarely scale to real-world needs in the long run.
- Deep understanding of the customer problem: We believe that there are too many "Let’s apply AI to underserved market X" startups that don’t really understand how to generate value for a particular target customer. We, therefore, systematically leverage our large angel investor community and industry network to quickly evaluate what real market needs are and how a startup might fit in.
- Identifying promising talent: Since we’re an early-stage investor, there is often little tangible traction data to guide an investment decision. We have defined a clear set of criteria we’re looking for when evaluating a startup and its founding team. Up until very recently, you needed a PhD in computer science or engineering to found an AI startup, but with the diffusion of technology, that is rapidly changing.
The Generative AI Space
It is easy to forget that generative AI is by no means a new phenomenon. AI models that produce content of some kind or interact with users have been around for a long time. Understandably, the proliferation of useful solutions such as Chat-GPT has awakened mainstream interest and alongside it, the appetite of investors. On generative AI applications, we have broken down the market at a very high level along the following segments:
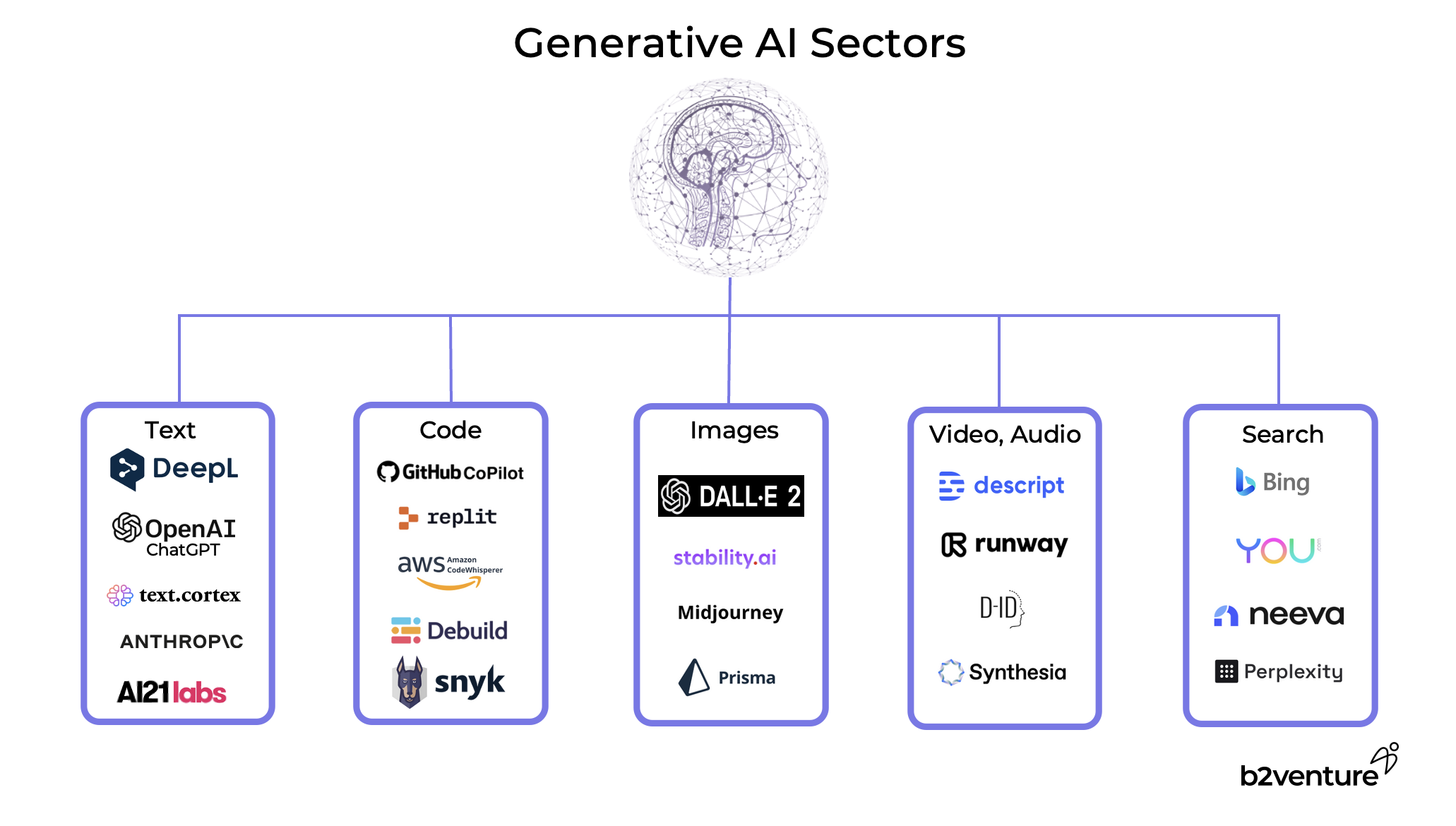
We find that frameworks are a great heuristic for discussing our investment approach, and we have developed a simple stack diagram for the generative AI space:
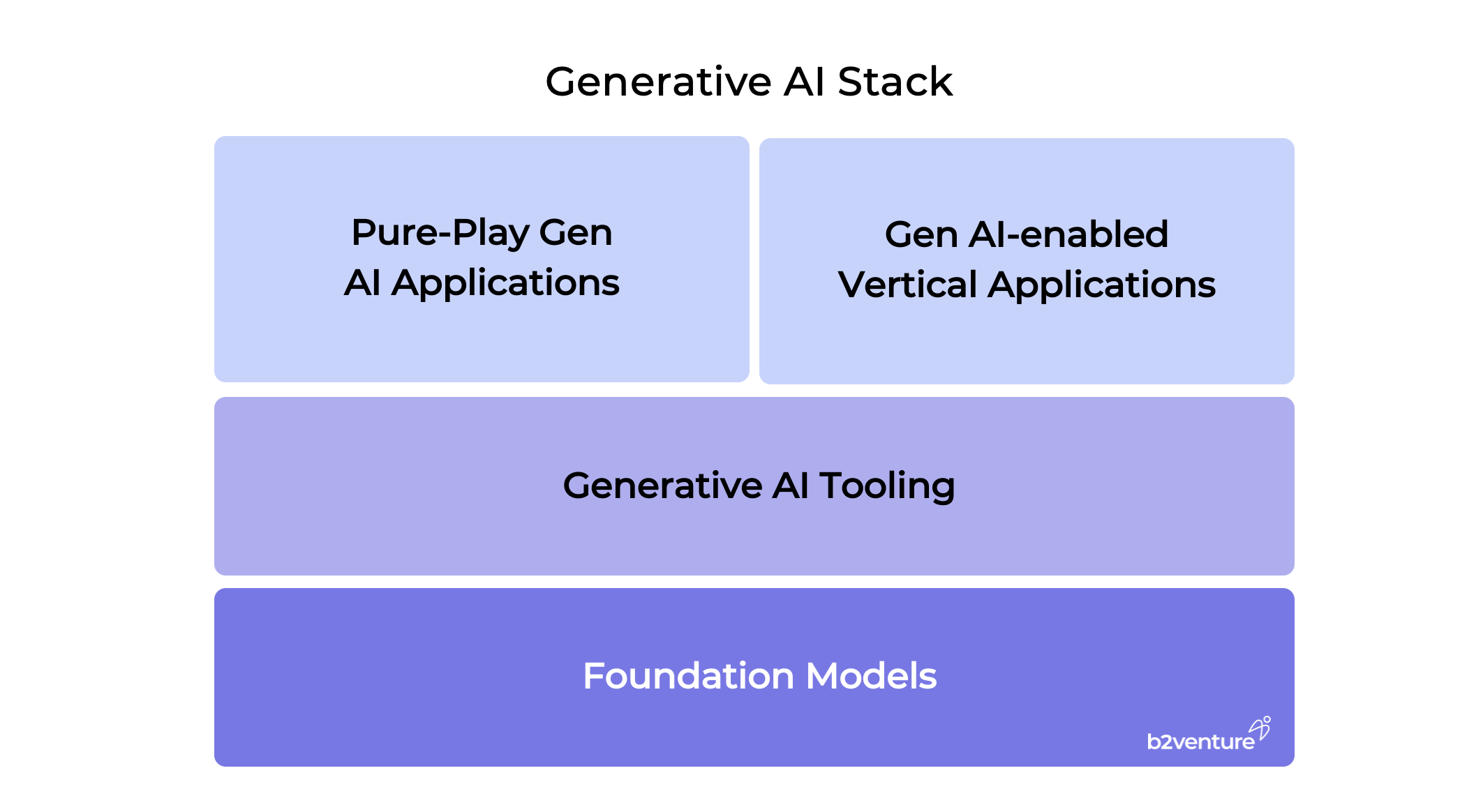
The genuinely new element in generative AI are powerful foundation models such as GPT-4 that cover a wide range of use cases. This has enabled a new generation of AI products and companies that don’t necessarily need to own the underlying models, but can build on readily available functionalities.
Two Scenarios: Oligopolistic vs Open Markets
When thinking about investment strategies in generative AI, a fundamental question is whether you believe in a more oligopolistic structure for the underlying foundation model platforms (similar to the mobile internet or Web 2.0, where only two platforms matter) or in an open model where many open and closed models could co-exist simultaneously (similar to the desktop internet or Web 1.0). The outcome will not only determine the right strategies for foundation model companies, but also the structure of the overall market for generative AI. We found it instructive to look at two extreme scenarios:
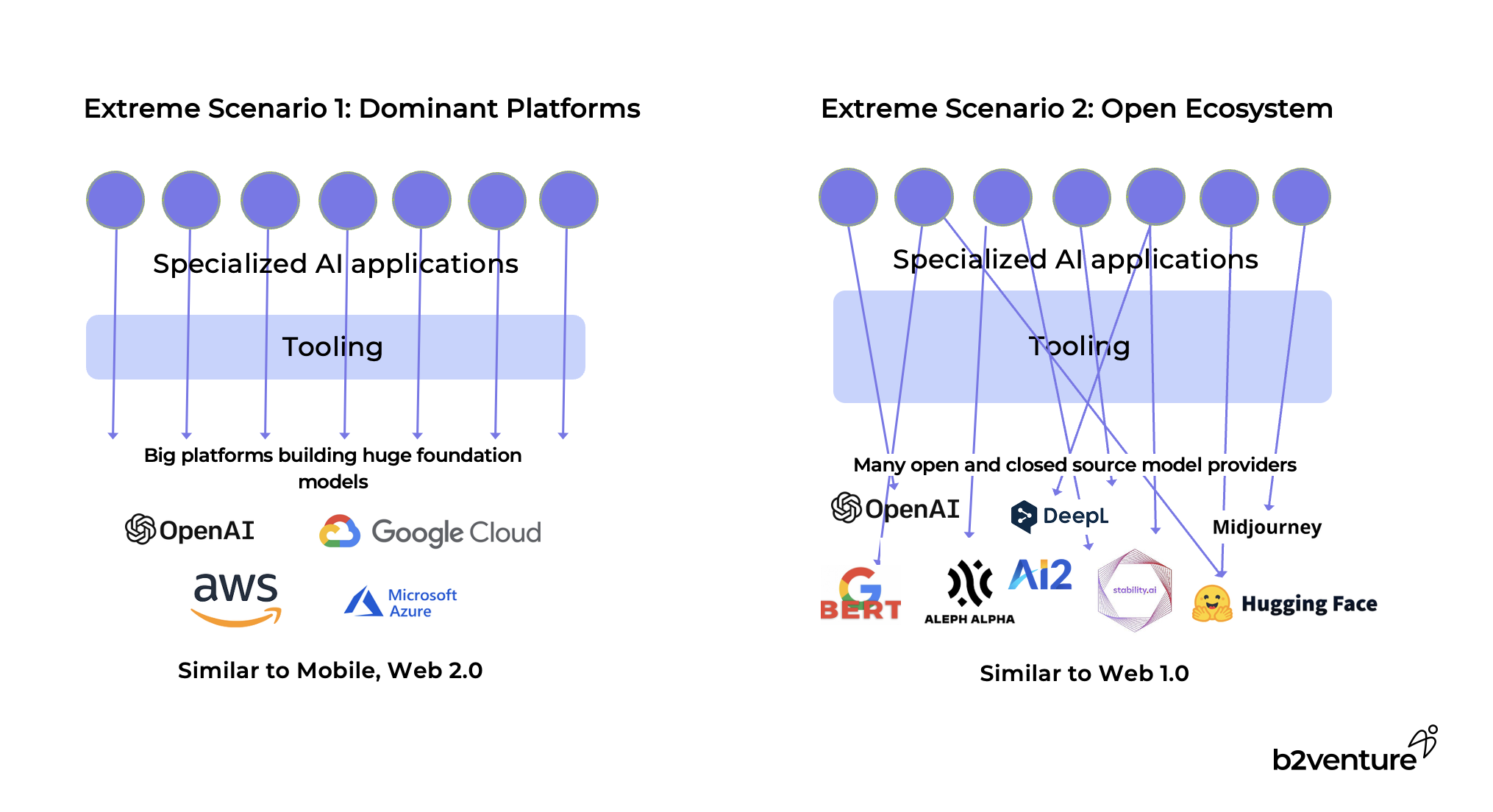
The first scenario, an oligopolistic structure for foundational models based on a few dominant platforms, would mean that investments in foundation models are going to be unsuccessful for almost everybody, since the incumbent tech giants would have an unassailable advantage with their colossal resources and infrastructure.
In this world, there would also be limited opportunities for tooling companies as well, since strong incumbents typically tend to build out complete solutions covering the entire stack (see, for instance, Microsoft’s recent announcement of its strategy for data management, analytics and AI with Fabric).
Application companies that build on top of these stacks would, of course, have room to provide specialized solutions for their target markets. However, the lessons from the mobile world will presumably apply here as well: as an application company, you are playing in someone else’s walled garden with their permission. If the platform providers don’t like your application, it’s absolutely possible that they will pull the plug and cut you off from essential services.
Furthermore, large platform owners frequently move up the stack and provide vertical solutions of their own in the most attractive segments, taking away market share from startups. This scenario is only desirable for the incumbent big tech firms. It would significantly hamper the creation and growth of new companies in the space.
The second scenario, an open world of permissionless innovation with many foundation models, would enable company creation on all layers up and down the stack and therefore create a richer, larger, more flexible market.
This view presumes there is a lot of room for innovation on the foundation model layer. Many signs are pointing towards the need for specialized models that excel at particular tasks and are more efficient than huge general-purpose models. The success of tools such as Stable Diffusion and Midjourney demonstrate that leading models can be built by very small, focused teams that often release their products as open source.
This rich environment would require a substantial layer of specialized tooling, since independent model companies are unlikely to be able to provide a rich stack of functionality. Application companies would be able to pick and choose models and tools to build their vertical applications. It’s likely that the successful and creative integration of several underlying elements will be part of the moat that these companies can build over time.
Our Hope for Generative AI: An Open Hybrid Market Model
As investors, we are very much in favor of an open market scenario. As a historical analogy, compare the very substantial value creation of the open World Wide Web with giant companies such as Google, Amazon and Meta versus the limited value creation of the mobile space where the largest app companies, such as Snap, Spotify and Zynga, have stayed smaller by over an order of magnitude.
Since building the very cutting edge of foundation models and particularly running the necessary infrastructure for inference is still going to be very expensive, we assume that we will see an open hybrid model, maybe comparable to what we’ve been seeing in cloud computing. Cloud infrastructure is dominated by a handful of huge hyperscalers, but there is still plenty of space for more specialized providers, including a very lively open source scene. One layer above pure infrastructure, there is plenty of room to build very large companies that embrace native capabilities, such as Snowflake, for example, which was arguably the first truly cloud-native data warehouse.
Back in 2017, Nvidia CEO Jensen Huang predicted a "Cambrian explosion of autonomous machines'', and the growth of specialized hardware, software, and machine learning models over the last several years have indeed led to an abundance of tools and technologies we associate with "artificial intelligence".
While many in the tech world have been interested in AI for a long time, the immense popularity of ChatGPT has kicked off a tremendous new wave of investment in AI startups and enormous interest in generative AI and large language models (LLMs).
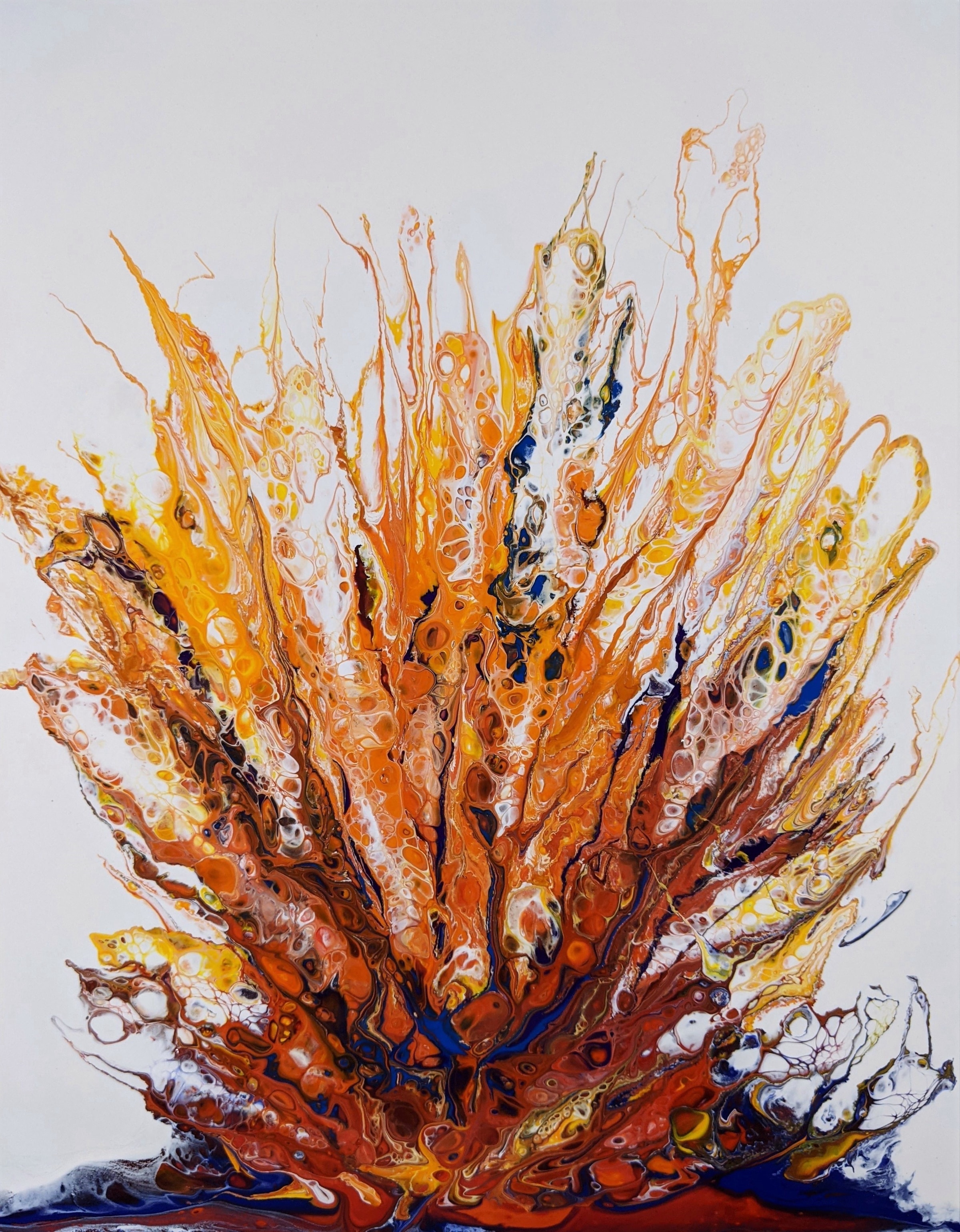
As one of the most active AI investors in Europe, b2venture has been investing in AI-related companies for a long time when we made our first investment in the translation engine DeepL – from today’s perspective, one of the very first generative AI companies - and since then a host of others, including LatticeFlow, Chattermill, Textcortex, Calvin Risk, Neptune AI, and Decentriq.
Over the coming months, we will be publishing our thoughts on how we see the market on AI developing (including the occasional experiment with new and emergent AI tools, such as Andreas Goeldi’s post about AI and venture capital.).
Our Approach
Historically, our approach to AI has been based on several dimensions:
- Long-term momentum: we are interested in sectors within AI that are fundamentally enabling technologies and will therefore see sustained growth over an extended period.
- Competitive intensity and structure: we evaluate the nature of competition within different subsectors of AI and try to understand how the market is structured, considering factors such as the number of players, market share, and barriers to entry. A key consideration is the role of incumbents that might adopt new AI technologies, as currently seen in the cases of Microsoft and Adobe.
- Business model opportunities: We consider various business models, such as open-source (where code is freely available and needs to be monetized indirectly) versus closed source (proprietary code that is sold as a product), analyzing the advantages and disadvantages of these different approaches.
- Defensibility: We assess the level of technical difficulty and the barriers to entry that could create a competitive advantage or "moat" for certain subsectors. Higher technical difficulty often offers more defensibilty and better long-term prospects, but comes at the price of requiring more capital and often longer adoption curves.
- Avoiding short-term trends (JOMO - "Joy of Missing Out"): we deliberately avoid short-term trends that may be popular but have a higher likelihood of failure in the long run. One example is "no-code" tools and platforms that allow people to create applications without coding, as these have demonstrable shortcomings and rarely scale to real-world needs in the long run.
- Deep understanding of the customer problem: We believe that there are too many "Let’s apply AI to underserved market X" startups that don’t really understand how to generate value for a particular target customer. We, therefore, systematically leverage our large angel investor community and industry network to quickly evaluate what real market needs are and how a startup might fit in.
- Identifying promising talent: Since we’re an early-stage investor, there is often little tangible traction data to guide an investment decision. We have defined a clear set of criteria we’re looking for when evaluating a startup and its founding team. Up until very recently, you needed a PhD in computer science or engineering to found an AI startup, but with the diffusion of technology, that is rapidly changing.
The Generative AI Space
It is easy to forget that generative AI is by no means a new phenomenon. AI models that produce content of some kind or interact with users have been around for a long time. Understandably, the proliferation of useful solutions such as Chat-GPT has awakened mainstream interest and alongside it, the appetite of investors. On generative AI applications, we have broken down the market at a very high level along the following segments:
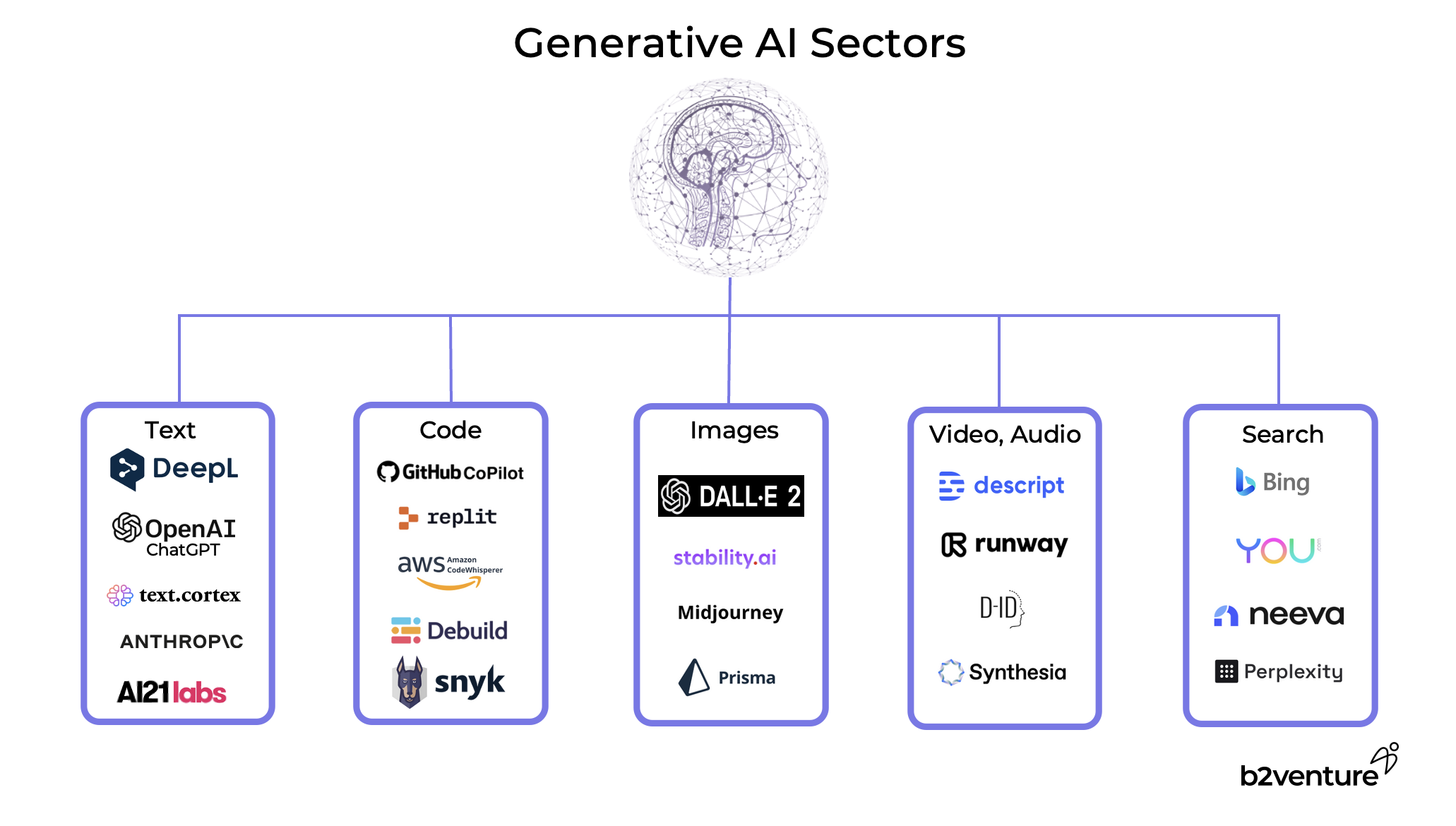
We find that frameworks are a great heuristic for discussing our investment approach, and we have developed a simple stack diagram for the generative AI space:
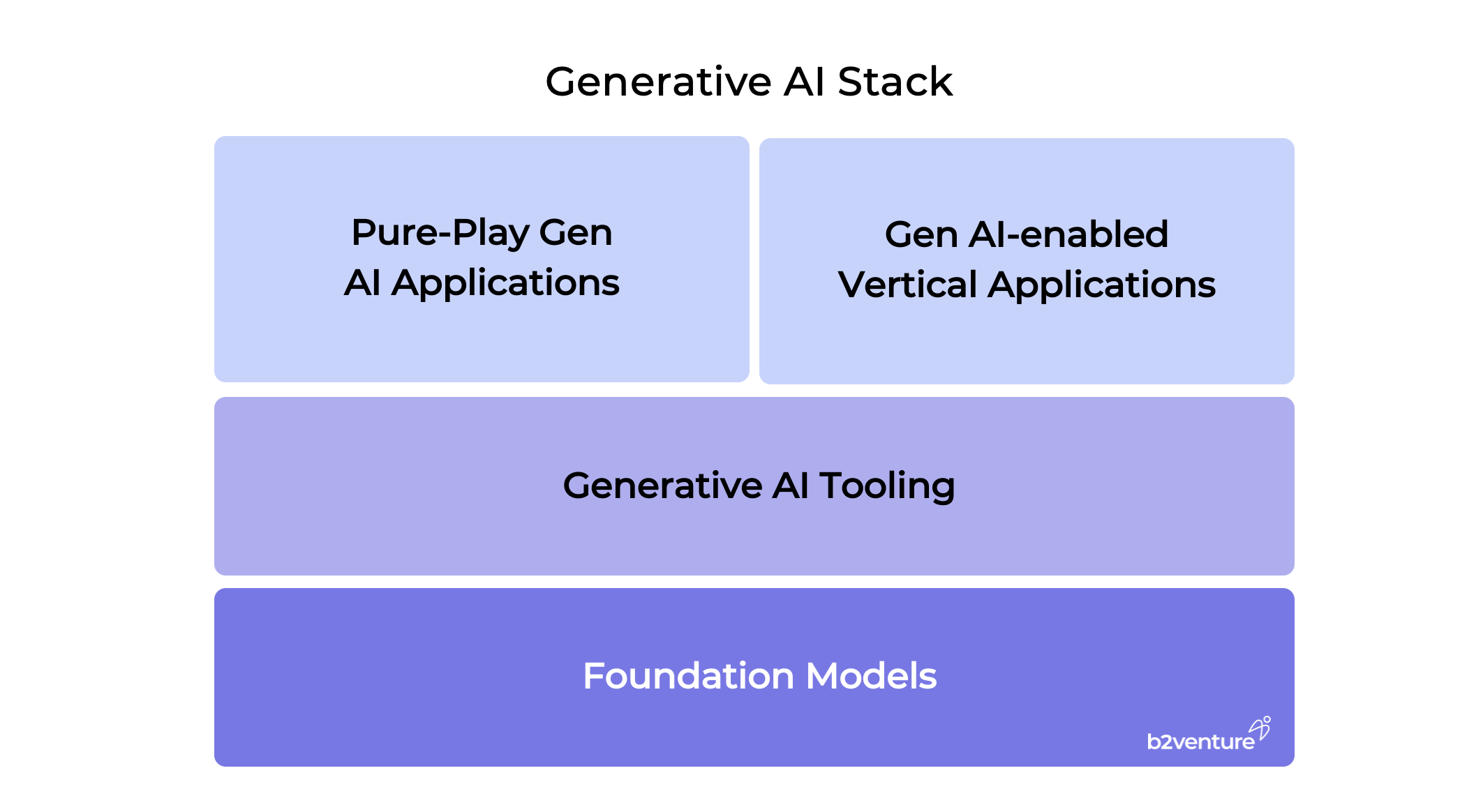
The genuinely new element in generative AI are powerful foundation models such as GPT-4 that cover a wide range of use cases. This has enabled a new generation of AI products and companies that don’t necessarily need to own the underlying models, but can build on readily available functionalities.
Two Scenarios: Oligopolistic vs Open Markets
When thinking about investment strategies in generative AI, a fundamental question is whether you believe in a more oligopolistic structure for the underlying foundation model platforms (similar to the mobile internet or Web 2.0, where only two platforms matter) or in an open model where many open and closed models could co-exist simultaneously (similar to the desktop internet or Web 1.0). The outcome will not only determine the right strategies for foundation model companies, but also the structure of the overall market for generative AI. We found it instructive to look at two extreme scenarios:
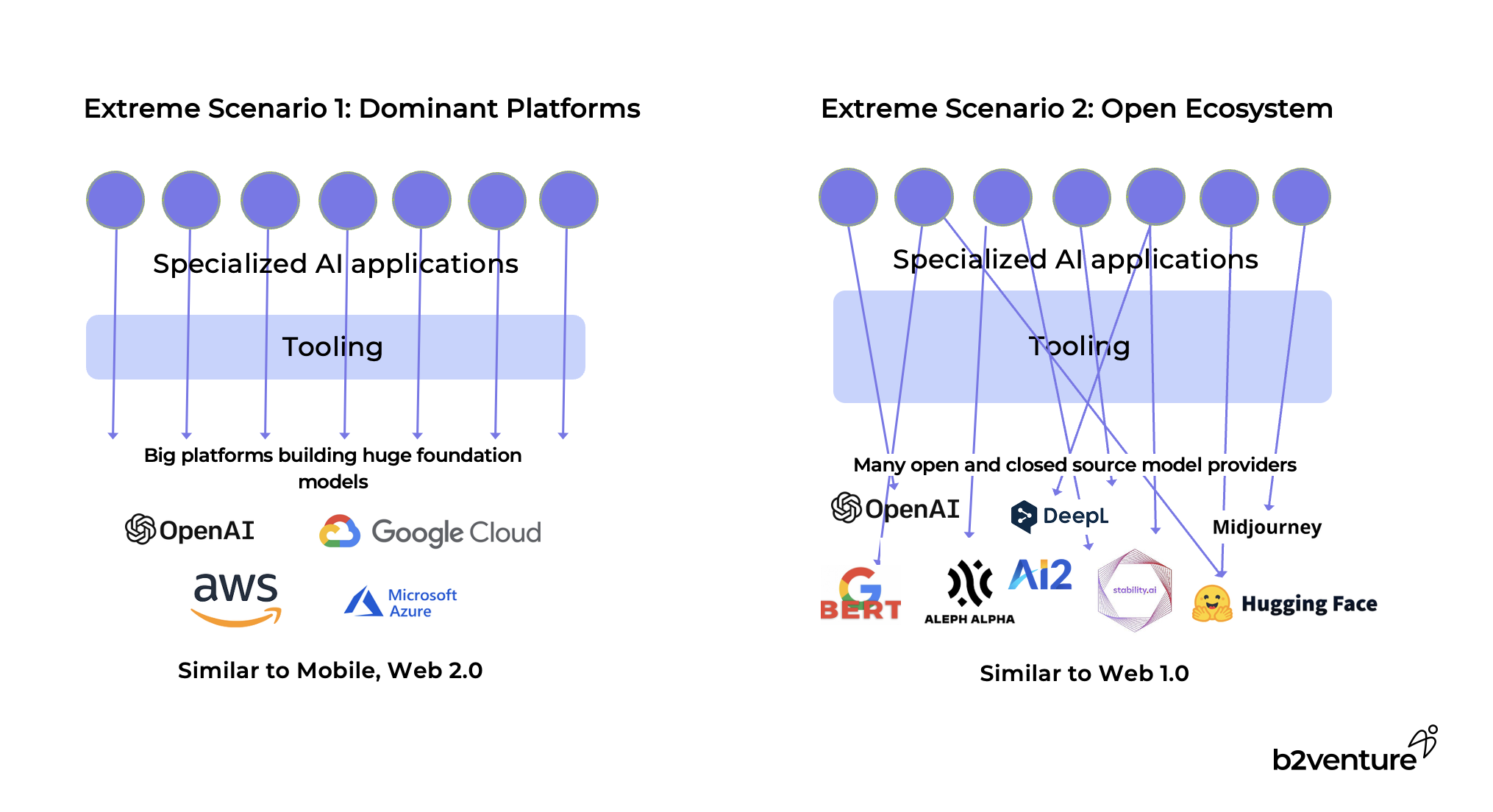
The first scenario, an oligopolistic structure for foundational models based on a few dominant platforms, would mean that investments in foundation models are going to be unsuccessful for almost everybody, since the incumbent tech giants would have an unassailable advantage with their colossal resources and infrastructure.
In this world, there would also be limited opportunities for tooling companies as well, since strong incumbents typically tend to build out complete solutions covering the entire stack (see, for instance, Microsoft’s recent announcement of its strategy for data management, analytics and AI with Fabric).
Application companies that build on top of these stacks would, of course, have room to provide specialized solutions for their target markets. However, the lessons from the mobile world will presumably apply here as well: as an application company, you are playing in someone else’s walled garden with their permission. If the platform providers don’t like your application, it’s absolutely possible that they will pull the plug and cut you off from essential services.
Furthermore, large platform owners frequently move up the stack and provide vertical solutions of their own in the most attractive segments, taking away market share from startups. This scenario is only desirable for the incumbent big tech firms. It would significantly hamper the creation and growth of new companies in the space.
The second scenario, an open world of permissionless innovation with many foundation models, would enable company creation on all layers up and down the stack and therefore create a richer, larger, more flexible market.
This view presumes there is a lot of room for innovation on the foundation model layer. Many signs are pointing towards the need for specialized models that excel at particular tasks and are more efficient than huge general-purpose models. The success of tools such as Stable Diffusion and Midjourney demonstrate that leading models can be built by very small, focused teams that often release their products as open source.
This rich environment would require a substantial layer of specialized tooling, since independent model companies are unlikely to be able to provide a rich stack of functionality. Application companies would be able to pick and choose models and tools to build their vertical applications. It’s likely that the successful and creative integration of several underlying elements will be part of the moat that these companies can build over time.
Our Hope for Generative AI: An Open Hybrid Market Model
As investors, we are very much in favor of an open market scenario. As a historical analogy, compare the very substantial value creation of the open World Wide Web with giant companies such as Google, Amazon and Meta versus the limited value creation of the mobile space where the largest app companies, such as Snap, Spotify and Zynga, have stayed smaller by over an order of magnitude.
Since building the very cutting edge of foundation models and particularly running the necessary infrastructure for inference is still going to be very expensive, we assume that we will see an open hybrid model, maybe comparable to what we’ve been seeing in cloud computing. Cloud infrastructure is dominated by a handful of huge hyperscalers, but there is still plenty of space for more specialized providers, including a very lively open source scene. One layer above pure infrastructure, there is plenty of room to build very large companies that embrace native capabilities, such as Snowflake, for example, which was arguably the first truly cloud-native data warehouse.
The Author
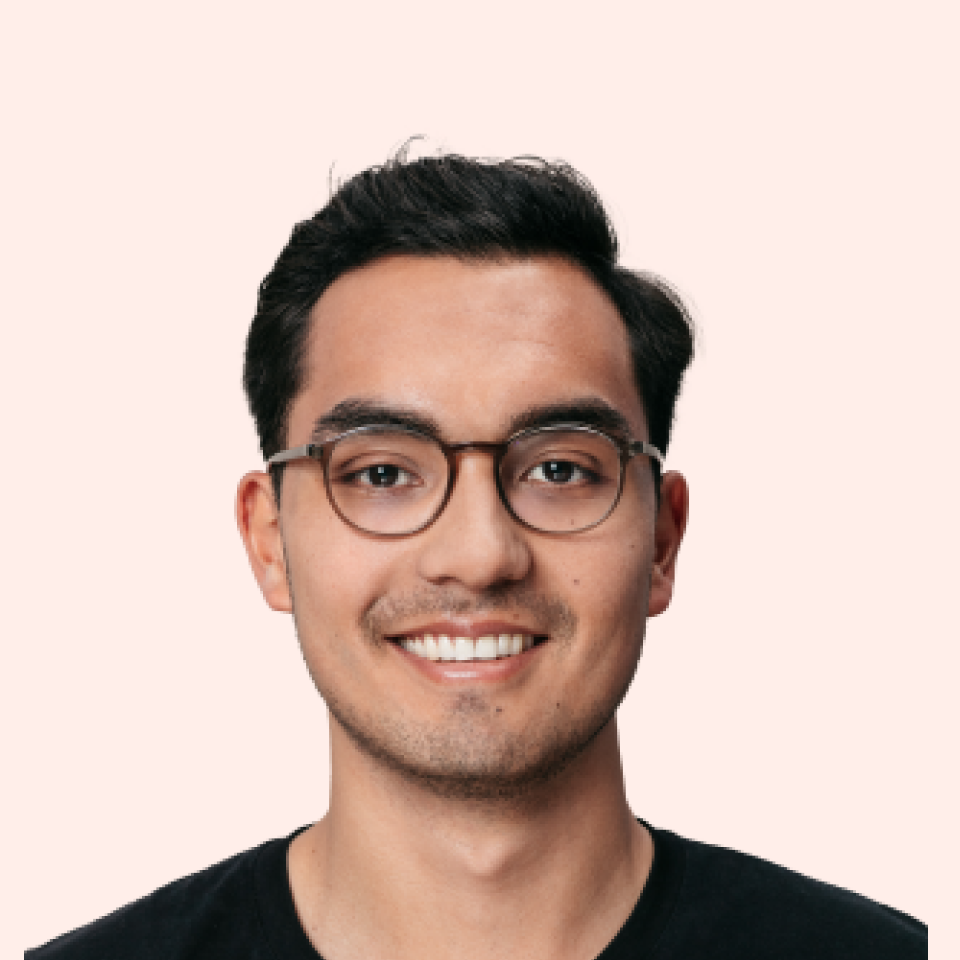
Damian Zaker
Associate
Damian Zaker joined the b2venture Fund Team in April of 2022, after previous stints at Visionaries Club and the CVC Division of the Swiss Stock Exchange.
Team