The Fourth Wave: How AI is Reshaping Software
The Fourth Wave: How AI is Reshaping Software

Are we at the beginning of a new era in software with AI?
Beyond the hype and exuberance of the last few years in both the public’s imagination and by investors, it seems like we're indeed on the cusp of AI leading to a new paradigm in software. This new paradigm, which could be described as a “fourth wave” of innovation in the software industry, is reshaping the underlying business models of software companies and promises to radically redefine the landscape of software development and consumption.
From Mainframes to AI: The Four Waves of the Software Industry
While AI will be the driver of the next major transformation in technology, it’s important to understand the historical context of this paradigm shift and where we are today. The software industry has evolved through distinct epochs, each characterized by technological advancements and shifts in business models. Each wave has followed a progressively shorter lifecycle, reflecting the acceleration of technological advancement and increasing speed of adoption:
- The Mainframe Era (1960s - 1980s): During this period, computing systems were large and centralized, with software and hardware sold as integrated packages. This era was primarily dominated by large vendors such as IBM and Digital Equipment due to the high cost and complexity of mainframe systems. Independent software vendors were struggling to build their businesses in these monolithic environments.
- The PC Era (late 1970s - 1990s): Marked by the proliferation of personal computers, this era democratized computing through reduced hardware costs and the development of desktop applications. The PC Era opened opportunities for independent software vendors, leading to the creation of a true software industry. Dominant companies such as Microsoft, Adobe and Oracle were started during this era.
- The SaaS Era (late 1990s - 2010s): Enabled by the growth of the internet and cloud computing, Software as a Service (SaaS) introduced subscription-based pricing models, centralized hosting, and enhanced user accessibility. This wave significantly altered how software was delivered, as well as providing a clear and attractive monetization model for companies to scale. SaaS led to a Cambrian explosion of the software industry. According to some estimates, there are now about 27,000 active SaaS companies. The SaaS model with its strong predictability and substantial initial capital needs has been beneficial for the VC industry as well, leading to the boom of the past decade.
- The AI Era (2022 - present & future): Enhanced capabilities through Large Language Models (LLMs) mark a paradigm shift in the way software will be created and used. We are witnessing a second Cambrian explosion in the software industry driven by AI.
The Fourth Wave: Four Possible Models
The emergence of AI presents new possibilities that challenge traditional business models, especially those of the most recent SaaS Era. Early market trends suggest four possible modalities for the fourth wave that could play out in the coming years:
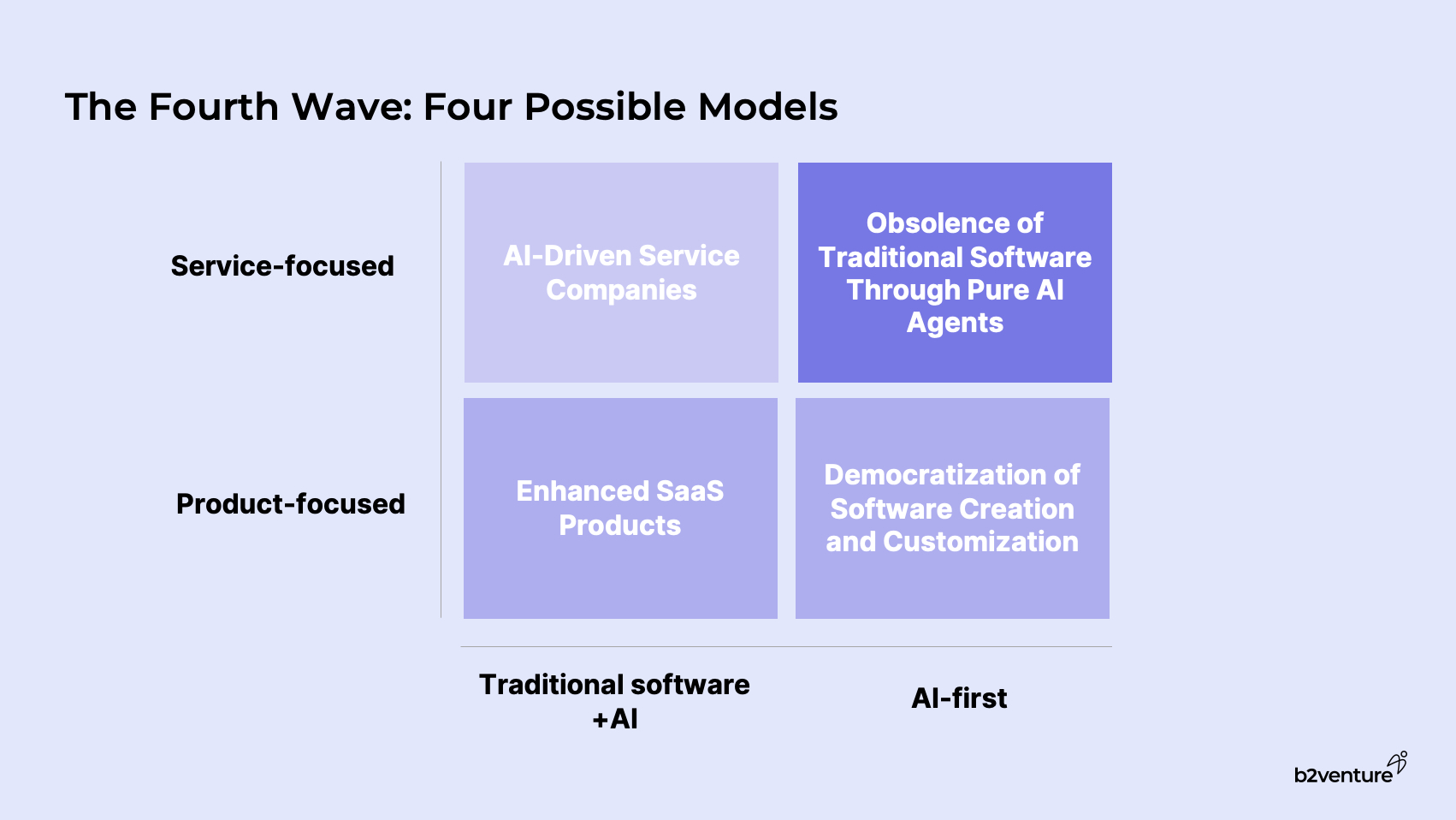
Model 1: Enhanced SaaS Products
AI will be integrated into existing SaaS offerings to boost efficiency and customer value, without fundamentally altering the SaaS business model. This involves AI-driven improvements such as automated customer support, predictive analytics, personalized user interfaces, and complex task automation. We see this already happening - our portfolio company Demoboost, for example, automates product demos with a host of AI-based features.

Model 2: “No Code Tools on Steroids” - The Democratization of Software Creation and Customization
AI tools could empower non-technical users to develop and customize software solutions, potentially transforming the software development landscape. Putting software creation directly into the hands of experts with deep domain knowledge could enable the development of software for underserved niche markets that might not be viable for software companies to pursue.
For example, an environmental scientist using an AI-assisted tool could create a complex custom system for monitoring endangered species, pulling in data from sensors, satellites and other systems – a type of application that wouldn’t be commercially attractive for traditional SaaS vendors.

There could be massive potential for startups in hyper-specific vertical markets by either creating the applications themselves or by developing tools that empower end users. While small niches might be too limited to be commercially attractive, there could be a path to scale by aggregating similar niche solutions with a high degree of automation.
Model 3: AI-Driven Service Companies
Companies could leverage AI to offer specialized services that may disrupt existing consulting and outsourcing paradigms. By reducing the burden on customers to manage the software themselves, particularly for non-core tasks, companies would be selling a service and its work result instead of the software.

For example, an AI-enhanced law firm would be able to automate workflows such as document review, legal research, and client interactions and relationship management to streamline servicing clients. This could lead to a dramatic reduction in costs and an increase in speed.
However, a major challenge is that such service-oriented companies would be selling to customers who are used to buying on a project basis - and not on a recurring model - which might result in high customer acquisition and service costs. But, if this model were to succeed, the overall software market could become much, much bigger by expanding into services. The total global service market is estimated to have a volume of 10 trillion dollars annually, compared to about 650 billion for software alone. If AI-driven service companies could capture even a slice of this volume with higher levels of efficiency, they could become hugely profitable.
Model 4: Obsolescence of Traditional Software Through Pure AI Agents
Another thesis is that AI could completely change how we think about software, with AI models and agents becoming sophisticated enough to replace traditional user-driven software tools entirely. AI systems could be capable of performing complex tasks autonomously, eliminating the need for conventional software interfaces. Advanced AI systems could also be able to autonomously perform end-to-end tasks that traditionally required dedicated software applications.
Concrete examples include AI-based CRM assistants that autonomously manage customer interactions, or AI-driven project management assistants that organize tasks, set reminders, and adjust schedules, even invoking other AI agents, or an AI-powered customer support system that proactively addresses issues and reduces the need for dedicated helpdesk software.
Unprecedented Growth Opportunities & Unique Challenges for Startups
If history is any guide, the promise of a transformational technology such as AI is an outsized opportunity, with potential revenue growth likely exceeding that of established SaaS markets. Yet there are a multitude of challenges for startups building on this fourth wave of innovation, with incumbents positioning themselves to take advantage of AI through cutting-edge research, massive capex expenditures, and the possibilities for distribution across their established products and services. We’ve updated our thinking on the possible overarching market scenarios in the “fourth wave”:

The reality for most AI startups at the present stage are the following challenges:
- Smaller foundation model builders will struggle to compete against cash-rich tech giants
- B2B apps currently often get stuck in proof-of-concept stages at large corporations
- B2C apps face high churn rates as consumers experiment with new technologies
Startups will also need to integrate disparate, complex data sources and ensure data quality, while addressing data privacy concerns amid constantly evolving regulatory environments and aligning diverse stakeholders within organizations for cohesive AI adoption. There is also enormous uncertainty surrounding how to effectively monetize AI solutions and maintain steady revenue streams amidst these evolving software models. Startups will also face stiff competition from resource-abundant tech giants, and how to balance the significant initial development costs for AI models and applications with potential long-term returns is perhaps the largest unknown in the entire tech industry.

Successfully navigating this new landscape will require rapid adaptability, strategic foresight, and a deep understanding of both the technological capabilities and the market dynamics of AI. For startups, the key will be to identify unique value propositions that leverage AI's strengths while addressing real market needs. Established companies, too, must embrace this change or risk obsolescence. The integration of AI into existing products and services is no longer optional but a necessity for maintaining competitiveness.
Startup Financing in the Era of AI
For investors, the challenge lies in accurately assessing the potential of AI startups amidst a technological and business landscape that is evolving rapidly. We broke down our approach to investing in AI in another post last year, but it’s important to highlight that many factors involved in the calculus of a classic SaaS investment case will change in the era of AI. Whereas traditional SaaS businesses developed clear parameters that applied to capital needs, revenue, cashflow, risk, scalability and capital efficiency, investors in the AI sector are confronted with much more complexity.
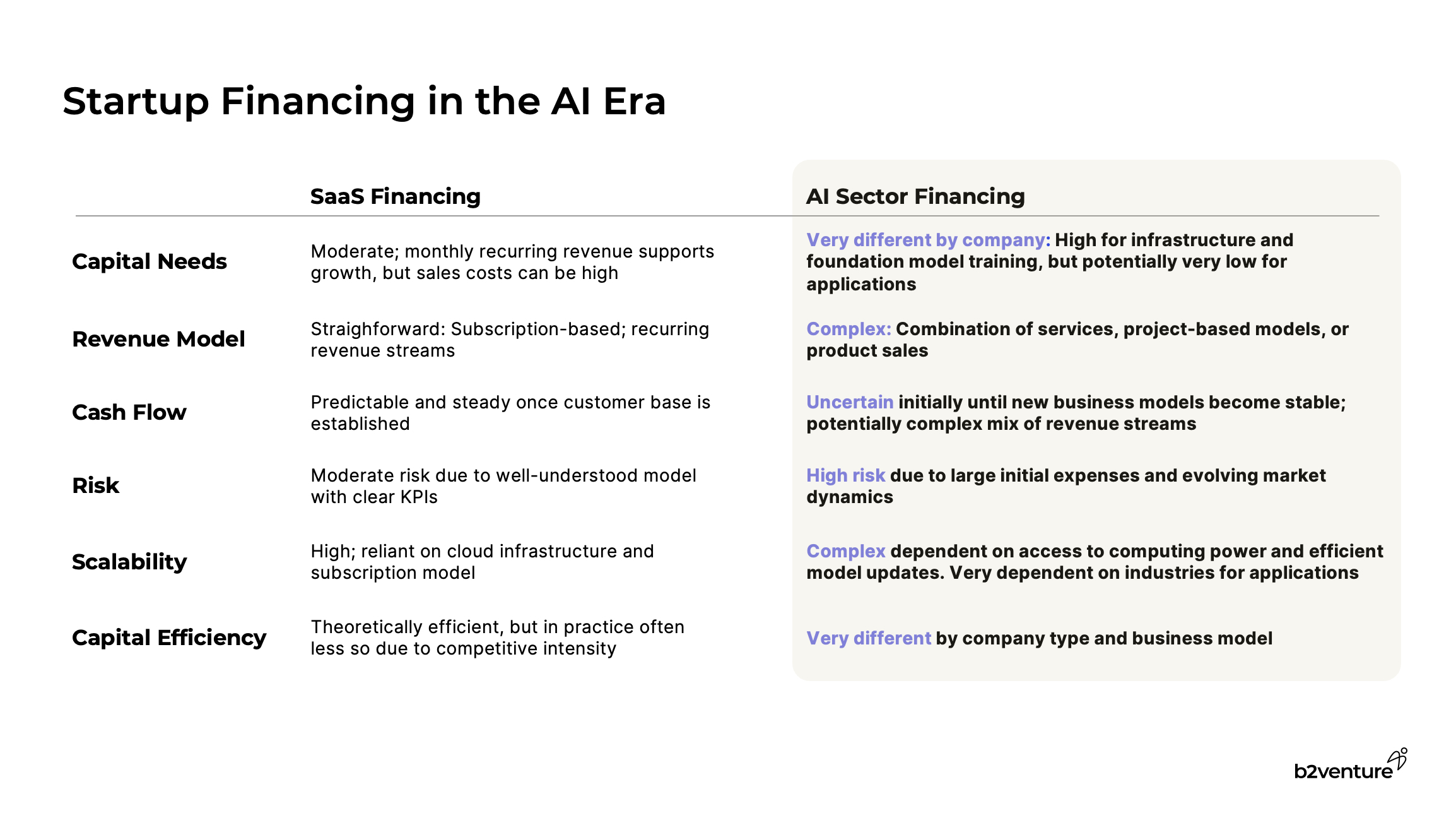
Traditional SaaS companies typically have moderate capital needs, straightforward subscription-based revenue, and predictable cash flow. AI companies, however, vary widely in capital requirements and might have more complex revenue models that are just starting to evolve. SaaS companies face moderate risk due to a well-understood model with clear KPIs, whereas AI companies face higher risks due to large initial expenses and evolving market dynamics. Scalability for SaaS companies is high, relying on cloud infrastructure and subscription models. For AI companies, growing to scale is much more complex, dependent on access to computing power, model updates, and the industry sector. Capital efficiency in SaaS is theoretically high but often less so in practice due to competitive intensity. For AI companies, capital efficiency is highly variable, depending on the company type and business model.
As we stand at the beginning of this new fourth wave, there are many more questions than answers as to how AI will penetrate established markets and industries, as well as what business models will ultimately succeed, which makes it an exciting time to be an entrepreneur building in this space. That excitement extends to investors too, who will need to assess the growth potential of AI startups in an environment of increased uncertainty but also open ups incredible opportunities for new approaches that can harness this new technology effectively and efficiently.

Are we at the beginning of a new era in software with AI?
Beyond the hype and exuberance of the last few years in both the public’s imagination and by investors, it seems like we're indeed on the cusp of AI leading to a new paradigm in software. This new paradigm, which could be described as a “fourth wave” of innovation in the software industry, is reshaping the underlying business models of software companies and promises to radically redefine the landscape of software development and consumption.
From Mainframes to AI: The Four Waves of the Software Industry
While AI will be the driver of the next major transformation in technology, it’s important to understand the historical context of this paradigm shift and where we are today. The software industry has evolved through distinct epochs, each characterized by technological advancements and shifts in business models. Each wave has followed a progressively shorter lifecycle, reflecting the acceleration of technological advancement and increasing speed of adoption:
- The Mainframe Era (1960s - 1980s): During this period, computing systems were large and centralized, with software and hardware sold as integrated packages. This era was primarily dominated by large vendors such as IBM and Digital Equipment due to the high cost and complexity of mainframe systems. Independent software vendors were struggling to build their businesses in these monolithic environments.
- The PC Era (late 1970s - 1990s): Marked by the proliferation of personal computers, this era democratized computing through reduced hardware costs and the development of desktop applications. The PC Era opened opportunities for independent software vendors, leading to the creation of a true software industry. Dominant companies such as Microsoft, Adobe and Oracle were started during this era.
- The SaaS Era (late 1990s - 2010s): Enabled by the growth of the internet and cloud computing, Software as a Service (SaaS) introduced subscription-based pricing models, centralized hosting, and enhanced user accessibility. This wave significantly altered how software was delivered, as well as providing a clear and attractive monetization model for companies to scale. SaaS led to a Cambrian explosion of the software industry. According to some estimates, there are now about 27,000 active SaaS companies. The SaaS model with its strong predictability and substantial initial capital needs has been beneficial for the VC industry as well, leading to the boom of the past decade.
- The AI Era (2022 - present & future): Enhanced capabilities through Large Language Models (LLMs) mark a paradigm shift in the way software will be created and used. We are witnessing a second Cambrian explosion in the software industry driven by AI.
The Fourth Wave: Four Possible Models
The emergence of AI presents new possibilities that challenge traditional business models, especially those of the most recent SaaS Era. Early market trends suggest four possible modalities for the fourth wave that could play out in the coming years:
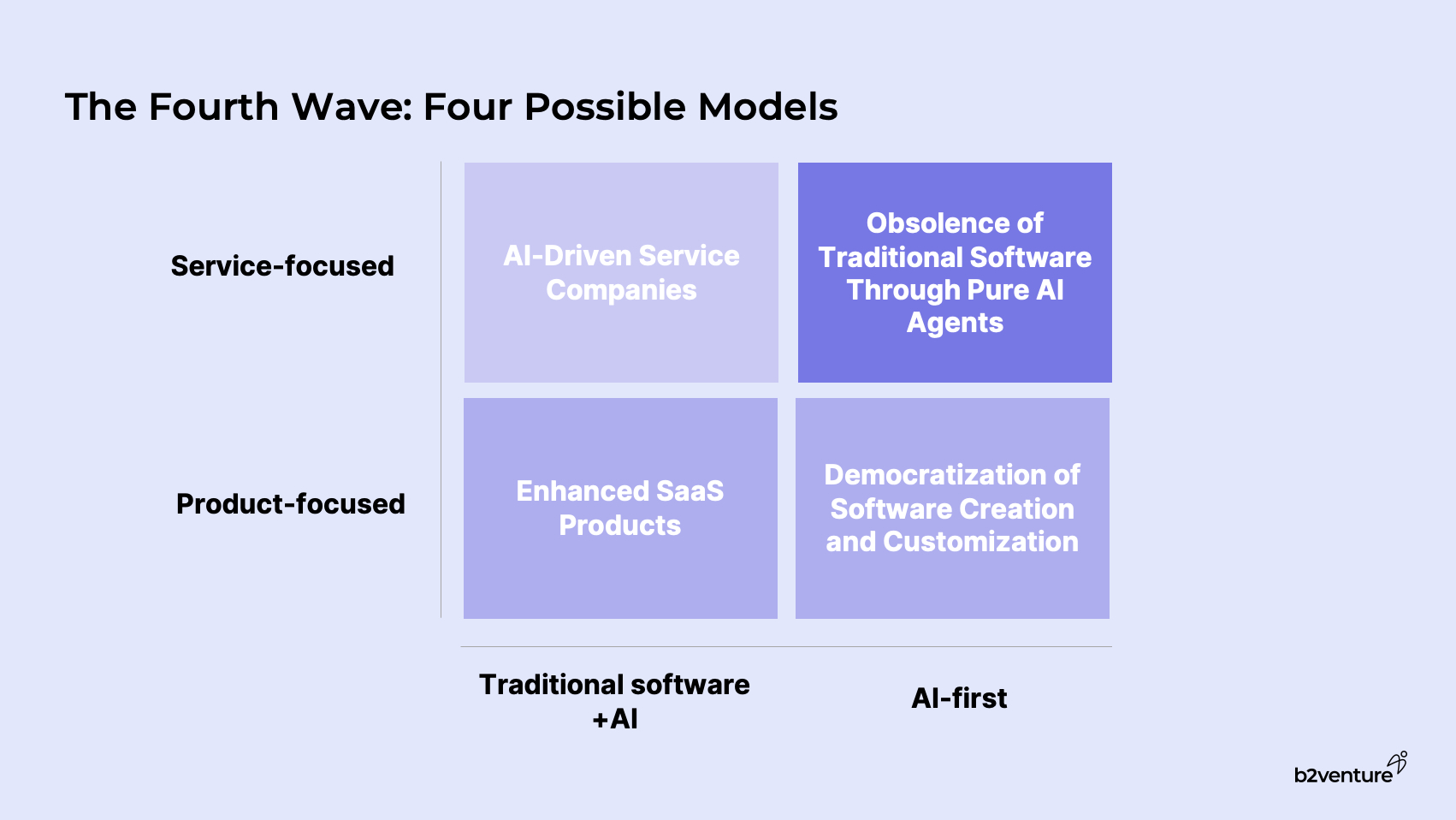
Model 1: Enhanced SaaS Products
AI will be integrated into existing SaaS offerings to boost efficiency and customer value, without fundamentally altering the SaaS business model. This involves AI-driven improvements such as automated customer support, predictive analytics, personalized user interfaces, and complex task automation. We see this already happening - our portfolio company Demoboost, for example, automates product demos with a host of AI-based features.

Model 2: “No Code Tools on Steroids” - The Democratization of Software Creation and Customization
AI tools could empower non-technical users to develop and customize software solutions, potentially transforming the software development landscape. Putting software creation directly into the hands of experts with deep domain knowledge could enable the development of software for underserved niche markets that might not be viable for software companies to pursue.
For example, an environmental scientist using an AI-assisted tool could create a complex custom system for monitoring endangered species, pulling in data from sensors, satellites and other systems – a type of application that wouldn’t be commercially attractive for traditional SaaS vendors.

There could be massive potential for startups in hyper-specific vertical markets by either creating the applications themselves or by developing tools that empower end users. While small niches might be too limited to be commercially attractive, there could be a path to scale by aggregating similar niche solutions with a high degree of automation.
Model 3: AI-Driven Service Companies
Companies could leverage AI to offer specialized services that may disrupt existing consulting and outsourcing paradigms. By reducing the burden on customers to manage the software themselves, particularly for non-core tasks, companies would be selling a service and its work result instead of the software.

For example, an AI-enhanced law firm would be able to automate workflows such as document review, legal research, and client interactions and relationship management to streamline servicing clients. This could lead to a dramatic reduction in costs and an increase in speed.
However, a major challenge is that such service-oriented companies would be selling to customers who are used to buying on a project basis - and not on a recurring model - which might result in high customer acquisition and service costs. But, if this model were to succeed, the overall software market could become much, much bigger by expanding into services. The total global service market is estimated to have a volume of 10 trillion dollars annually, compared to about 650 billion for software alone. If AI-driven service companies could capture even a slice of this volume with higher levels of efficiency, they could become hugely profitable.
Model 4: Obsolescence of Traditional Software Through Pure AI Agents
Another thesis is that AI could completely change how we think about software, with AI models and agents becoming sophisticated enough to replace traditional user-driven software tools entirely. AI systems could be capable of performing complex tasks autonomously, eliminating the need for conventional software interfaces. Advanced AI systems could also be able to autonomously perform end-to-end tasks that traditionally required dedicated software applications.
Concrete examples include AI-based CRM assistants that autonomously manage customer interactions, or AI-driven project management assistants that organize tasks, set reminders, and adjust schedules, even invoking other AI agents, or an AI-powered customer support system that proactively addresses issues and reduces the need for dedicated helpdesk software.
Unprecedented Growth Opportunities & Unique Challenges for Startups
If history is any guide, the promise of a transformational technology such as AI is an outsized opportunity, with potential revenue growth likely exceeding that of established SaaS markets. Yet there are a multitude of challenges for startups building on this fourth wave of innovation, with incumbents positioning themselves to take advantage of AI through cutting-edge research, massive capex expenditures, and the possibilities for distribution across their established products and services. We’ve updated our thinking on the possible overarching market scenarios in the “fourth wave”:

The reality for most AI startups at the present stage are the following challenges:
- Smaller foundation model builders will struggle to compete against cash-rich tech giants
- B2B apps currently often get stuck in proof-of-concept stages at large corporations
- B2C apps face high churn rates as consumers experiment with new technologies
Startups will also need to integrate disparate, complex data sources and ensure data quality, while addressing data privacy concerns amid constantly evolving regulatory environments and aligning diverse stakeholders within organizations for cohesive AI adoption. There is also enormous uncertainty surrounding how to effectively monetize AI solutions and maintain steady revenue streams amidst these evolving software models. Startups will also face stiff competition from resource-abundant tech giants, and how to balance the significant initial development costs for AI models and applications with potential long-term returns is perhaps the largest unknown in the entire tech industry.

Successfully navigating this new landscape will require rapid adaptability, strategic foresight, and a deep understanding of both the technological capabilities and the market dynamics of AI. For startups, the key will be to identify unique value propositions that leverage AI's strengths while addressing real market needs. Established companies, too, must embrace this change or risk obsolescence. The integration of AI into existing products and services is no longer optional but a necessity for maintaining competitiveness.
Startup Financing in the Era of AI
For investors, the challenge lies in accurately assessing the potential of AI startups amidst a technological and business landscape that is evolving rapidly. We broke down our approach to investing in AI in another post last year, but it’s important to highlight that many factors involved in the calculus of a classic SaaS investment case will change in the era of AI. Whereas traditional SaaS businesses developed clear parameters that applied to capital needs, revenue, cashflow, risk, scalability and capital efficiency, investors in the AI sector are confronted with much more complexity.
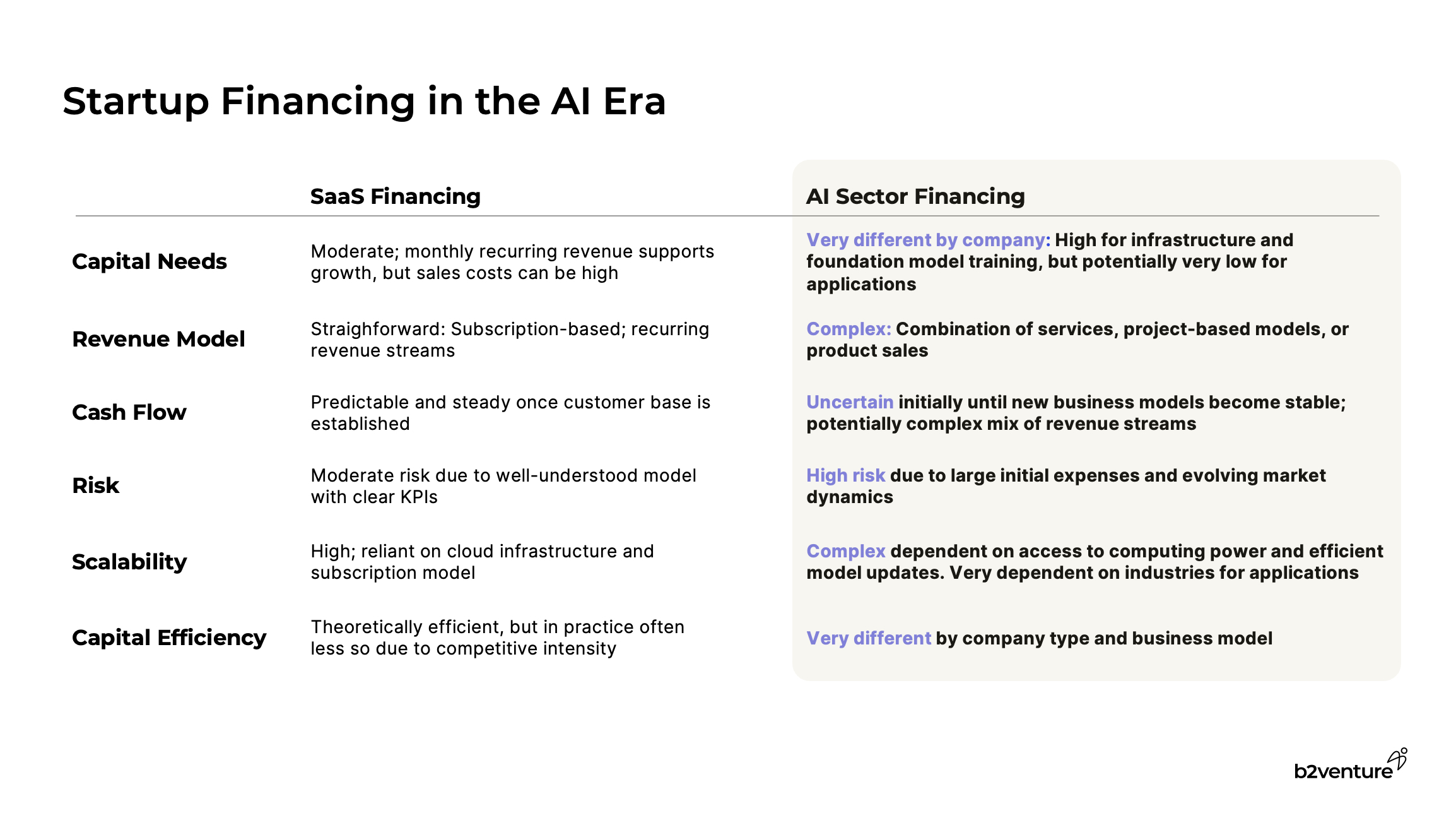
Traditional SaaS companies typically have moderate capital needs, straightforward subscription-based revenue, and predictable cash flow. AI companies, however, vary widely in capital requirements and might have more complex revenue models that are just starting to evolve. SaaS companies face moderate risk due to a well-understood model with clear KPIs, whereas AI companies face higher risks due to large initial expenses and evolving market dynamics. Scalability for SaaS companies is high, relying on cloud infrastructure and subscription models. For AI companies, growing to scale is much more complex, dependent on access to computing power, model updates, and the industry sector. Capital efficiency in SaaS is theoretically high but often less so in practice due to competitive intensity. For AI companies, capital efficiency is highly variable, depending on the company type and business model.
As we stand at the beginning of this new fourth wave, there are many more questions than answers as to how AI will penetrate established markets and industries, as well as what business models will ultimately succeed, which makes it an exciting time to be an entrepreneur building in this space. That excitement extends to investors too, who will need to assess the growth potential of AI startups in an environment of increased uncertainty but also open ups incredible opportunities for new approaches that can harness this new technology effectively and efficiently.
The Author
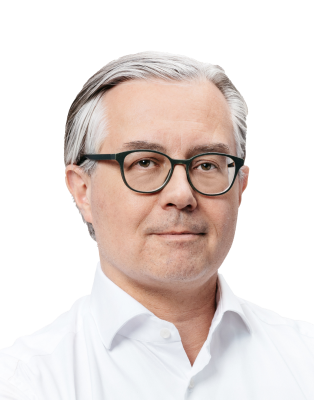
Andreas Goeldi
Partner
Andreas Goeldi is Partner and has been part of the b2venture Fund Team since 2019. He is an avid technologist, serial entrepreneur, and investor with over 25 years’ experience.
Team