5 ways to think about investing in Generative AI in 2023 and beyond
5 ways to think about investing in Generative AI in 2023 and beyond
Over the last several months, generative AI models such as ChatGPT and DALL-E have captured the public imagination. And with underlying drivers such as increasing computing power and the benefits of scale coming to bear, we are beginning to see the first glimmers of how generative AI could have a broader impact.
At b2venture we have long been focused on the promise of machine learning and AI as a catalyst for new, cutting-edge businesses. One of our first investments in the area was in 2009 with DeepL, which recently announced a financing round by IVP, Atomico, Bessemer and WiL. In 2023, we are poised to see a lot more happening in the space in the near future. Therefore we wanted to highlight five areas we think could have an outsized impact and provide a framework for how we think about AI and a new generation of startups that could be the major players of tomorrow (including our own landscape of the space below).
1. Intelligent Automation Tools disrupting Low Code and No-code analytics
Low code solutions have been the holy grail of software development for a long time and have gained new credence with the growing shortage of skilled workers. A challenge for all low-code tools is the lack of flexibility: for the tech team, low-code ready-made solutions can work in the beginning when creating a new software product but are difficult to scale once the team reaches a certain size. For business operations, the analytics of low-code environments can be limiting as well — use cases such as analyzing customer behavior, for example, requires a level of granularity and flexibility that low-code tools seldom provide. For that reason from a strategic investment perspective we are more interested in AI-enabled automation tools that allow companies to build data pipelines, and automation workflows, and to enrich these automated processes with additional code.
2. Hypergrowth with Open Source
We think there is ample space for startups building on Open Source Software (OSS) solutions in the AI space — although at first glance it might appear more difficult to monetize, since the company is essentially giving away large parts of its code base for free.
Nevertheless, OSS can be the best business model for rapid growth since it incentivizes third party developers to interact with a company’s codebase and contribute to it, thereby using crowd-intelligence in order to improve the product and also serving as an entry point for product led growth.
One interesting example — though outside the horizontal AI sector — is in the data pipe lining tool space, where Airbyte has been chipping away customers from Fivetran and other incumbents via the power of an open source play.
Particularly in the space of cutting edge AI models, the most interesting and most innovative models are frequently made available to the public domain after a short period. For example, Alphafold, a deep learning model developed for protein structure prediction, has been open-sourced — anyone can access and use it without limitations. Open-source implementations of models for text, image, and audio generation are widely made available — for example via the Hugging Face Transformer Library which contains open-source implementations of models such as GPT.
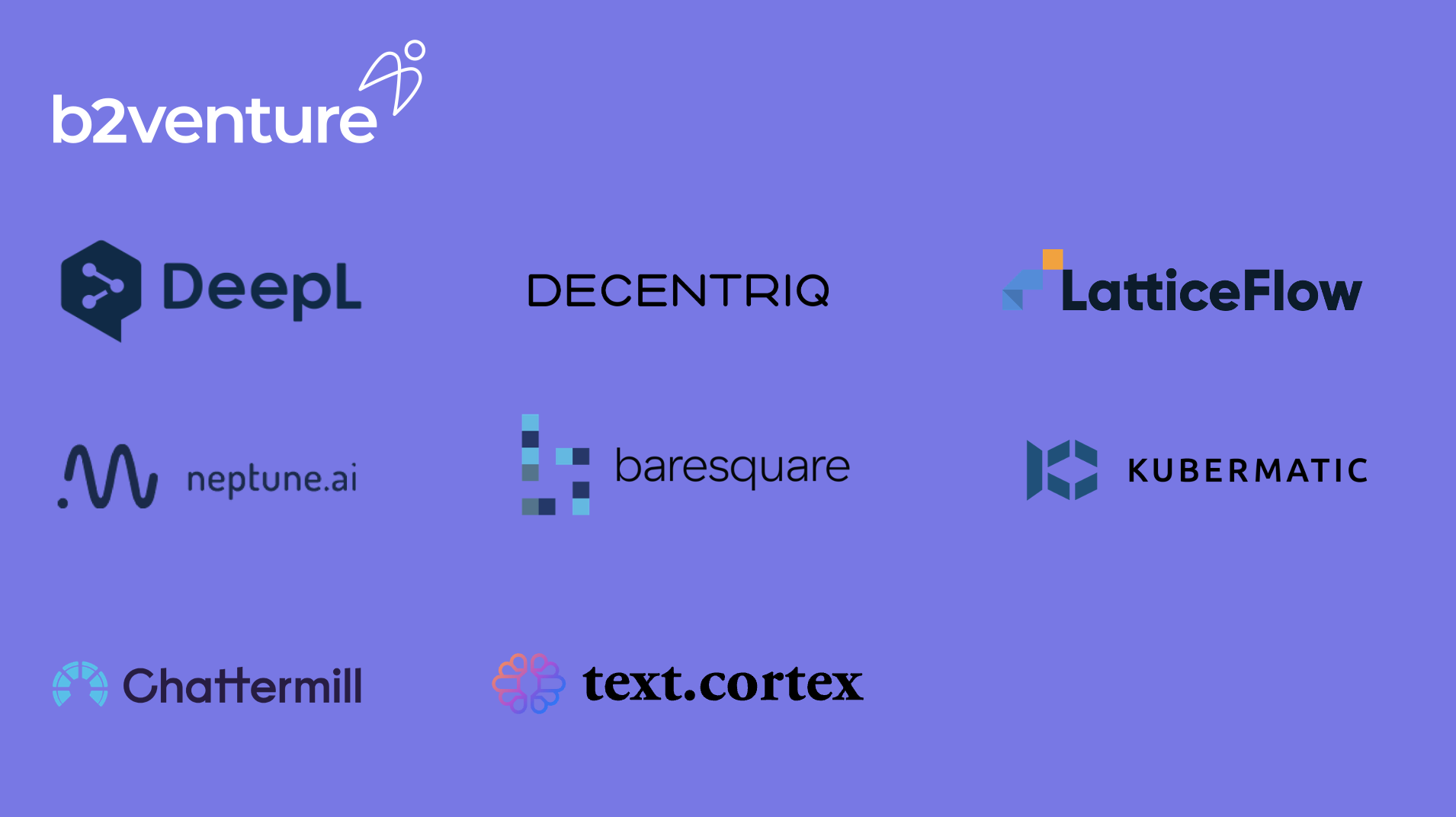
3. Small AI: Training Hard vs. Training Smart
AI has a massive appetite for computation power. The recent AI boom has been fuelled partially by using more and more compute — which not only comes with high training costs — with OpenAI spending for example $12 million for a single GPT-3 training run — but also escalating energy consumption and CO2 emissions.
Fortunately, there are smarter, more environmentally friendly and less costly approaches. Instead of training essentially similar general purpose models with more and more parameters, startups can focus on “verticalized” models. These models are not only more sustainable, but offer best-in-class results for bespoke purposes. One example from the b2venture portfolio is TextCortex. This Browser Extension easily makes high-performing specialized models available for copywriting and content-creation.
Moreover— as mentioned above — deploying open-source implementations of the latest models also can avoid escalating training costs for AI startups.
4. Generative AI — User Layers above the Tech Stack
Another framework we use to think about business potential of AI targets are the top-most layers of the tech stack of any company — i.e. the layers that non-tech people use. Generative AI models here have the potential to disrupt numerous visual and semantic workflows.
Generating textual content and especially copywriting has been the first field to be transformed, but in the future all kinds of textual and non-textual documents will be generated in a way which relies on AI. Examples are Large-Language-Models which output written content and are transforming workflows which seemed unthinkable to automate, such as writing business plans, user stories, financial documents and even computer programs. And there is a high demand to automatically turn data into insights on queries such as ‘Which employee sold the most last Christmas?’
Moreover, Text-to-image models such as Stable Diffusion, Midjourney and Dall-E which use machine learning to generate unique pictures from simple text prompts, have captured the public imagination in recent months and are disrupting design workflows. The future also promises the generation of visual and semantic components at once — eg. for the creation of slide decks or designing user interfaces. Next level personal assistants which nudge us in the right direction are also emerging. Moreover, as a recent report by McKinsey Quantum Black points out, AI is set to revolutionize fields which are highly interactive, such as customer service. This will be the case because Large-Language-Models are able to approximate human behavior closely. Finally, many physical workflows such as photo shootings can be replaced by AI generated photos.
Most of these workflows will require a human-in-the-loop to sanity check the AI generated products. Automated filters are improving, but for the moment are still easy to circumvent. For example, by asking indirectly, by means of prompting the model for a computer program or a story in which a character asks the actual question, the models will freely deliver unethical results. Moreover, there is a problem of “hallucinations” — replies which are factually inaccurate but seem correct. Companies like Calvin Risk can help companies to mitigate the risks of deploying AI.
And here without further ado, our generative AI market map — the full list with more details and additional startups (which did not make it on the list) can be shared on request.
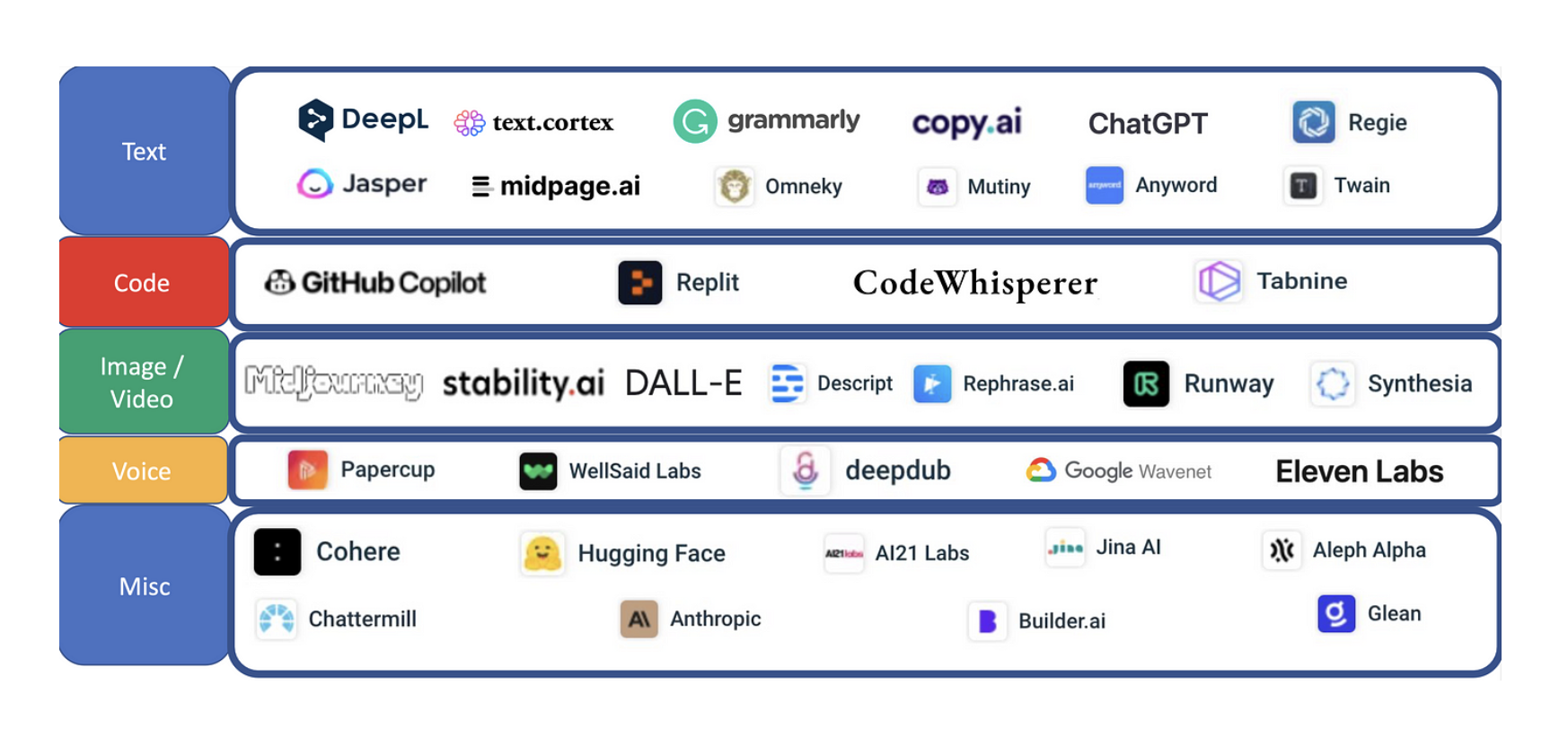
5. “Data is not the new oil”: Data Observability and Quality to build robust AI models
As Tim O’Reilly wrote last year: “Data is not the new oil. It is the new sand. Like silicon, which makes up 28% of the earth’s crust, it is ubiquitous and is only made valuable by an enormous set of industrial-scale processes.” AI models used in production must rely on carefully crafted data, otherwise they could turn out to be snake oil — and for that purpose companies need access to high-performance computing resources such as Kubernetes Clusters provided by Kubermatic. And as important — companies have to sustain a pipeline of high quality data.
This is a challenge much more easily explained than achieved; in many companies, for example, there are multiple data pipelines for the same information, and developing consistent pipelines or single sources of truth remain elusive. Many enterprises even lack an overview about what data is available and in which silos it lives, whether it’s trustworthy and how to make the best use of it. Therefore, a major market opportunity exists to provide tools and interfaces that provide data catalogs and data observability dashboards, which allow AI and data science teams to build on trustworthy data.
Working with sensible data such as in Marketing and Pharmaceutical industries requires the use of secure data rooms and confidential computing such as provided by Decentriq.
We believe artificial intelligence is at a critical juncture. The pace of innovation is accelerating faster than ever. Machine learning models are now being pushed out of the development lab and into everyday operations, even in more traditional companies, which is why topics like the sustainability, robustness or trustworthiness of AI are becoming increasingly important.
There are currently a lot of exciting startups on the move here that are bringing new approaches to the market and making AI really suitable for everyday use. b2venture portfolio companies such as LatticeFlow and Neptune.ai are at the forefront of making AI more applicable, and we are very excited to see what the coming year has in store.
Over the last several months, generative AI models such as ChatGPT and DALL-E have captured the public imagination. And with underlying drivers such as increasing computing power and the benefits of scale coming to bear, we are beginning to see the first glimmers of how generative AI could have a broader impact.
At b2venture we have long been focused on the promise of machine learning and AI as a catalyst for new, cutting-edge businesses. One of our first investments in the area was in 2009 with DeepL, which recently announced a financing round by IVP, Atomico, Bessemer and WiL. In 2023, we are poised to see a lot more happening in the space in the near future. Therefore we wanted to highlight five areas we think could have an outsized impact and provide a framework for how we think about AI and a new generation of startups that could be the major players of tomorrow (including our own landscape of the space below).
1. Intelligent Automation Tools disrupting Low Code and No-code analytics
Low code solutions have been the holy grail of software development for a long time and have gained new credence with the growing shortage of skilled workers. A challenge for all low-code tools is the lack of flexibility: for the tech team, low-code ready-made solutions can work in the beginning when creating a new software product but are difficult to scale once the team reaches a certain size. For business operations, the analytics of low-code environments can be limiting as well — use cases such as analyzing customer behavior, for example, requires a level of granularity and flexibility that low-code tools seldom provide. For that reason from a strategic investment perspective we are more interested in AI-enabled automation tools that allow companies to build data pipelines, and automation workflows, and to enrich these automated processes with additional code.
2. Hypergrowth with Open Source
We think there is ample space for startups building on Open Source Software (OSS) solutions in the AI space — although at first glance it might appear more difficult to monetize, since the company is essentially giving away large parts of its code base for free.
Nevertheless, OSS can be the best business model for rapid growth since it incentivizes third party developers to interact with a company’s codebase and contribute to it, thereby using crowd-intelligence in order to improve the product and also serving as an entry point for product led growth.
One interesting example — though outside the horizontal AI sector — is in the data pipe lining tool space, where Airbyte has been chipping away customers from Fivetran and other incumbents via the power of an open source play.
Particularly in the space of cutting edge AI models, the most interesting and most innovative models are frequently made available to the public domain after a short period. For example, Alphafold, a deep learning model developed for protein structure prediction, has been open-sourced — anyone can access and use it without limitations. Open-source implementations of models for text, image, and audio generation are widely made available — for example via the Hugging Face Transformer Library which contains open-source implementations of models such as GPT.
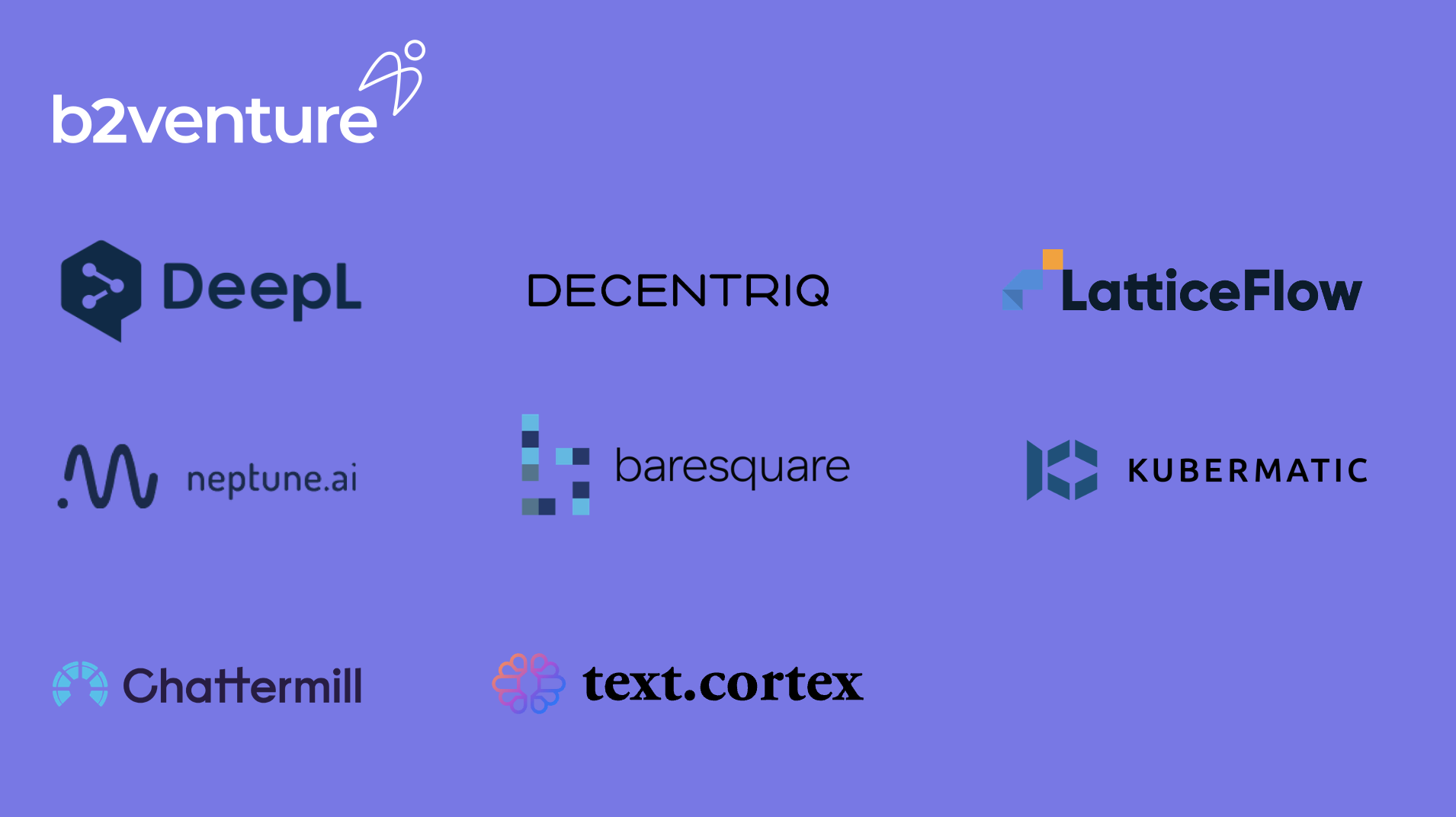
3. Small AI: Training Hard vs. Training Smart
AI has a massive appetite for computation power. The recent AI boom has been fuelled partially by using more and more compute — which not only comes with high training costs — with OpenAI spending for example $12 million for a single GPT-3 training run — but also escalating energy consumption and CO2 emissions.
Fortunately, there are smarter, more environmentally friendly and less costly approaches. Instead of training essentially similar general purpose models with more and more parameters, startups can focus on “verticalized” models. These models are not only more sustainable, but offer best-in-class results for bespoke purposes. One example from the b2venture portfolio is TextCortex. This Browser Extension easily makes high-performing specialized models available for copywriting and content-creation.
Moreover— as mentioned above — deploying open-source implementations of the latest models also can avoid escalating training costs for AI startups.
4. Generative AI — User Layers above the Tech Stack
Another framework we use to think about business potential of AI targets are the top-most layers of the tech stack of any company — i.e. the layers that non-tech people use. Generative AI models here have the potential to disrupt numerous visual and semantic workflows.
Generating textual content and especially copywriting has been the first field to be transformed, but in the future all kinds of textual and non-textual documents will be generated in a way which relies on AI. Examples are Large-Language-Models which output written content and are transforming workflows which seemed unthinkable to automate, such as writing business plans, user stories, financial documents and even computer programs. And there is a high demand to automatically turn data into insights on queries such as ‘Which employee sold the most last Christmas?’
Moreover, Text-to-image models such as Stable Diffusion, Midjourney and Dall-E which use machine learning to generate unique pictures from simple text prompts, have captured the public imagination in recent months and are disrupting design workflows. The future also promises the generation of visual and semantic components at once — eg. for the creation of slide decks or designing user interfaces. Next level personal assistants which nudge us in the right direction are also emerging. Moreover, as a recent report by McKinsey Quantum Black points out, AI is set to revolutionize fields which are highly interactive, such as customer service. This will be the case because Large-Language-Models are able to approximate human behavior closely. Finally, many physical workflows such as photo shootings can be replaced by AI generated photos.
Most of these workflows will require a human-in-the-loop to sanity check the AI generated products. Automated filters are improving, but for the moment are still easy to circumvent. For example, by asking indirectly, by means of prompting the model for a computer program or a story in which a character asks the actual question, the models will freely deliver unethical results. Moreover, there is a problem of “hallucinations” — replies which are factually inaccurate but seem correct. Companies like Calvin Risk can help companies to mitigate the risks of deploying AI.
And here without further ado, our generative AI market map — the full list with more details and additional startups (which did not make it on the list) can be shared on request.
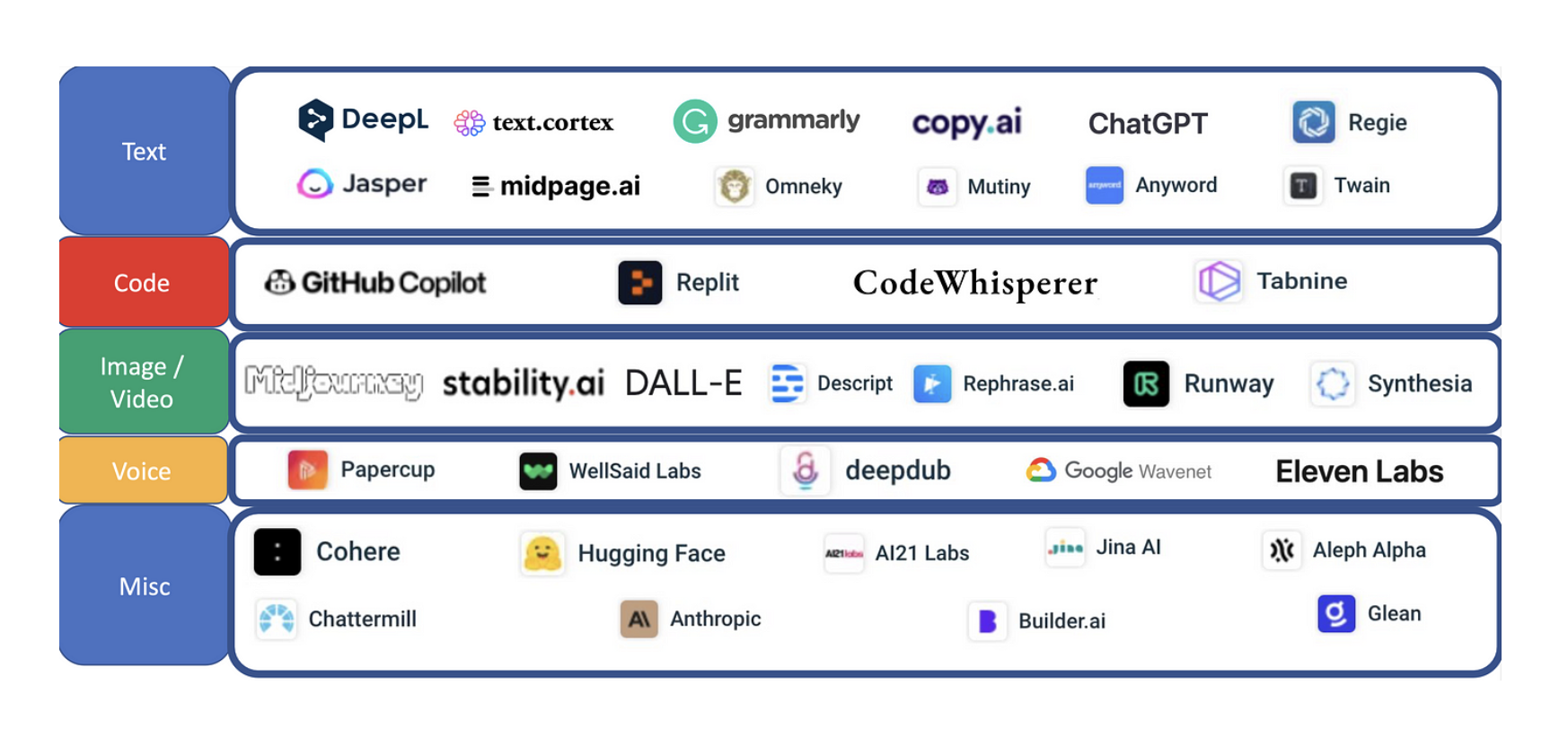
5. “Data is not the new oil”: Data Observability and Quality to build robust AI models
As Tim O’Reilly wrote last year: “Data is not the new oil. It is the new sand. Like silicon, which makes up 28% of the earth’s crust, it is ubiquitous and is only made valuable by an enormous set of industrial-scale processes.” AI models used in production must rely on carefully crafted data, otherwise they could turn out to be snake oil — and for that purpose companies need access to high-performance computing resources such as Kubernetes Clusters provided by Kubermatic. And as important — companies have to sustain a pipeline of high quality data.
This is a challenge much more easily explained than achieved; in many companies, for example, there are multiple data pipelines for the same information, and developing consistent pipelines or single sources of truth remain elusive. Many enterprises even lack an overview about what data is available and in which silos it lives, whether it’s trustworthy and how to make the best use of it. Therefore, a major market opportunity exists to provide tools and interfaces that provide data catalogs and data observability dashboards, which allow AI and data science teams to build on trustworthy data.
Working with sensible data such as in Marketing and Pharmaceutical industries requires the use of secure data rooms and confidential computing such as provided by Decentriq.
We believe artificial intelligence is at a critical juncture. The pace of innovation is accelerating faster than ever. Machine learning models are now being pushed out of the development lab and into everyday operations, even in more traditional companies, which is why topics like the sustainability, robustness or trustworthiness of AI are becoming increasingly important.
There are currently a lot of exciting startups on the move here that are bringing new approaches to the market and making AI really suitable for everyday use. b2venture portfolio companies such as LatticeFlow and Neptune.ai are at the forefront of making AI more applicable, and we are very excited to see what the coming year has in store.
The Author
Team