TechBio Market Map: AI in Drug Discovery and Development
TechBio Market Map: AI in Drug Discovery and Development
Right now, we are at the juncture of realizing we need the ability to engineer bio, and a fuller ability to engineer it (i.e., we’re still in the installation phase). In the tech industry, the analog period for the web led to the creation of massive companies like Amazon and Google. Given the power of the combined trends — and the scale of the challenges and massive market of healthcare — we should expect to similarly see the rise of a few potentially trillion-dollar companies at scale: the equivalent of a Bio GAFA, finally. Vijay Pande, Andreesen Horowitz

We’ve shared our thoughts on what is happening in the emerging space between medicine, the life sciences, and GenAI dubbed TechBio in a previous post. Now we are bringing a more detailed market map, zooming in on the software solutions, AI- and ML-driven companies, and foundational tech layers that are reshaping drug discovery and development.
Why should you read this? If you’re a founder, this is a chance to get inside the mind of an early-stage VC and understand what SaaS and technology investors look for when evaluating opportunities in TechBio, and use this knowledge (or terminology) to refine your next pitch. If you’re an investor, consider this your field guide to identifying key segments that deserve a closer look.
Our working definition of TechBio: these are technology platforms that don’t just complement scientific work but are inherently tied to advancing it—geared towards delivering an impactful end product. This spans everything from diagnostics to carbon capture. For this post we are focusing on the arena we think is best placed for disruptive growth: drug discovery and development.
How is the market evolving and which themes do we see?
To quote Patrick Möller, CIO of Bayer Pharma: „My personal bet is that AI will transform productivity for everyone in the pharma value chain by 2026.“ The conversation has moved beyond isolated success stories; it’s about rethinking how the entire industry works, from drug discovery to delivery. We’ve seen a surge of large language models (LLMs) tailored for biology, including AlphaFold cracking protein structures, Meta’s ESMFold, and BioGPT making waves in specialized research. The era of biology’s digitization is here, reinforced by the cloud’s power to virtualize samples and catalog findings like never before. The result is a massive explosion of data, boosted by the continuous advancements in DNA sequencing and analytics.
But despite the explosion of data — biopharma companies today collect seven times more investigational drug data than two decades ago — drug approval timelines have not gotten any shorter, nor have hit rates improved. The ecosystem is buzzing with different perspectives, approaches, and strategies. The value of public data? Hotly debated. Open-source models? Divisive. What is the right approach to collaboration and knowledge sharing? Still up for discussion. Whether building a structured data foundation or diving into the mess of unstructured data enhanced by LLMs: everyone is still trying to crack the code. Some, such as pharma giant Merck, start with a clear problem and build outward. Others have started by setting up AI taskforces that can end up feeling like solutions in search of a problem. And when it comes to building AI stacks, most companies are preferring to develop this critical part of the company in-house, as seen with AstraZeneca’s open-source model adoption and Qiagen’s data-to-knowledge graph pipeline.
Major Challenges
When evaluating the potential success of new TechBio companies, it’s worth considering not just the technology but also the characteristics of the market dynamics in which these companies are building. Large pharma organizations face the usual challenges — outdated infrastructure, entrenched processes, and the cultural shifts needed to embrace digital tech. Add to this the internal tug-of-war between commercial teams prioritizing boosting sales, research teams racing to complete trials, and healthcare practitioners and payors focusing on minimizing costs, and you will find different interests and goals inside of one organization.
When it comes to AI and ML, the hurdles are even higher. The data burden is real, especially with personalized medicine. Genomic data is coming from massive projects like UK Biobank, Genomics England, and All Of Us, each housed on its own clunky, proprietary platform. For small, scrappy teams, moving models from one dataset to another while ensuring reproducibility and maintaining security is a mammoth task.
And then there’s the complex question of trust. In AI, it’s not enough to say that it works—people want to know how it works. The proof is not in the pudding here; stakeholders need to understand what is in the recipe. Historically, drug research has always been a mix of science and mystery, from Fleming’s chance discovery of penicillin to the opaque leaps of combinatorial chemistry. Breakthroughs often relied on trial, error, and intuition. Today, AI revives that skepticism, with data and models operating beyond human intuition. It’s not just about results but ensuring stakeholders can trace the logic to something they trust.
Our TechBio Market Map
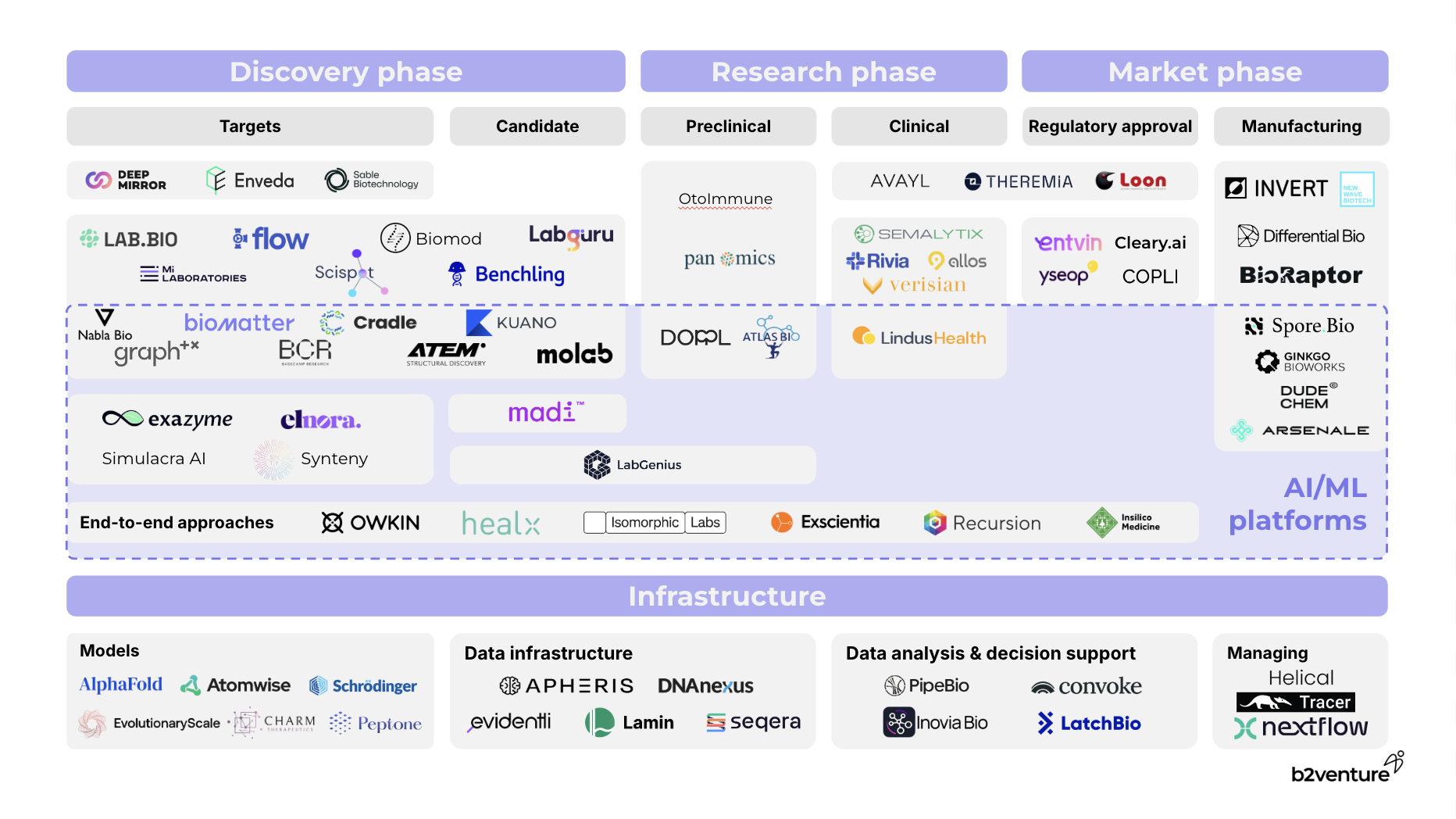
From a technology perspective, there are two major waves shaping the TechBio stack: Like the Modern Data Stack in tech (whose evolution Matt Turck has extensively explored here), the data infrastructure in life sciences is evolving and far from consolidated, echoing the early 2010s tech landscape where fragmented data solutions gradually coalesced into unified systems. Life sciences now face the challenge of integrating vast, multi-modal datasets — from genomic records to clinical trials — amidst legacy platforms, before any AI can sit on top of it. As a senior executive at Merck puts it, focusing on public data and robust infrastructure could allow the whole industry to “stand on the shoulders of giants.”
The second wave is the ML/AI innovation cycle, supercharged by the rise of LLMs and generative AI, like AlphaFold and Peptone, pushing predictive models to new heights. Compared to general tech, the relationship between data and AI in life sciences is even more deeply symbiotic — tight feedback loops are essential to validate hypotheses, guide better-targeted experiments, and optimize the selection of promising candidates, ultimately speeding up the path from discovery to development.
We find that the best way to think of the TechBio market map is as an assembly line — a continuum from discovery to development, and ultimately, value generation. Along this assembly line, we find three archetypes of TechBio companies:
- Applications (the top layer): SaaS-based platforms that provide outward-facing tools, catering to researchers and pharma companies to streamline operations of the assembly line.
- ML & AI platforms (the middle): This layer embodies the most comprehensive approach, where proprietary technology integrates seamlessly with wet lab operations, either in-house or through strategic partnerships. These platforms enable tight data feedback loops that accelerate learning cycles, bridging tech development and drug discovery. Some companies focus on specific parts of the assembly line (for now), like graphTX integrating advanced machine learning to optimize gene and cell therapy processes. Others are starting to develop an end-to-end stack, like Owkin leveraging AI models to identify novel drug targets and predict outcomes, and pipelines from first-generation TechBio companies such as Recursion that have progressed to clinical trials.
- Infrastructure (the foundation): These are the building blocks, essential for both pharma giants and startups striving for product quality. Companies like Benchling, Seqera, and Lamin Labs play pivotal roles in mining vast datasets and supporting seamless data replatforming and analysis. We’ve recently backed a team in this space - stay tuned for the announcement shortly.
Though each layer is crucial to propelling life sciences into its next era, we have a particular interest in the infrastructure layer and look forward to seeing more companies evolving in this part of the stack.
TechBio Business Models
The intersection of biotech and AI/ML technology brings a unique tension into business models, balancing two inherently conflicting worlds. BioTech traditionally centers around proprietary IP and asset development, tying value to a specific therapeutic with limited application. The binary risk of whether these assets work, tied to a long timeline, does not match the typical software VC fund profile tailored to platforms which gain traction over time. Meanwhile, technology-centric startups focus on market problems and scalable solutions, allowing for early-stage pivots—a crucial trait for growth.
AI is moving these two worlds closer together by accelerating R&D and promising faster asset development (and exits) as well as new, more scalable business strategies.
There’s a delicate decision to be made for a start-up between licensing their platform (aligning with the VC-friendly, scalable SaaS revenue model) and keeping the platform to itself to develop their own assets, which comes with significant capital needs but higher potential returns. Timing is key: early outlicensing can provide revenue and validate a company’s platform, while transitioning to in-house asset development can capture greater upside as the platform matures. For insights on platform validation and key KPIs, Pablo Lubroth’s TechBio KPIs guide is a must-read.
Which themes are we closely tracking for winners based in Europe?
While the US has deep VC pockets and aggressive M&A strategies to drive rapid late-stage development, Europe’s scientific talent is unmatched, with a network of world-renowned academic and research institutions fueling its strength in early-stage R&D. The European pharma landscape thrives on collaboration between public research institutions and private enterprises (which even US giants such as Eli Lilly are tapping into), creating a fertile environment for innovation. Success cases like Novo Nordisk show that it’s possible to capitalize on Europe’s collaborative spirit to become leaders in diabetes care and innovative treatments.
European startups excel in forming strategic partnerships with larger pharma players, as illustrated by Owkin, which collaborates with major companies to harness AI for precision medicine. The landscape is seeing an uptick in technologies spun out into commercial applications; in 2021, European TechBio startups raised $8.5bn, signaling that TechBio is starting to command a bigger slice of venture funding. Companies like LabGenius exemplify Europe’s capacity to build and scale impactful startups.
We are particularly interested in teams solving pivotal challenges with software-driven approaches:
- Trust: Driven by challenges outlined above around data scarcity and transparency how a ML/AI platform works, the European emphasis on scientific rigor and deep validation makes it fertile ground for startups that enable data transparency and the trustworthiness of ML/AI platforms.
- Data infrastructure: Europe’s collaborative ecosystem reveals the need to move beyond mere data collection (e.g., UK Biobank) and transform data into scientifically actionable insights. The key is quality over quantity—not just more models but cleaner, validated data that integrates experimental insights. Experimental data consistently outperforms silicon-generated data, and models thrive on exposure to failure (negative data) to sharpen their predictive power. Maintaining clean data, monitoring for drift, and ensuring reproducibility are essential.
- Lab automation: Given the tighter data feedback loops needed, this area is ripe for disruption, and we’ve not yet seen a larger wave of companies addressing this challenge.
If you’re a founder building in this space or an investor ready to back the next wave of TechBio innovation, let’s connect!
Right now, we are at the juncture of realizing we need the ability to engineer bio, and a fuller ability to engineer it (i.e., we’re still in the installation phase). In the tech industry, the analog period for the web led to the creation of massive companies like Amazon and Google. Given the power of the combined trends — and the scale of the challenges and massive market of healthcare — we should expect to similarly see the rise of a few potentially trillion-dollar companies at scale: the equivalent of a Bio GAFA, finally. Vijay Pande, Andreesen Horowitz

We’ve shared our thoughts on what is happening in the emerging space between medicine, the life sciences, and GenAI dubbed TechBio in a previous post. Now we are bringing a more detailed market map, zooming in on the software solutions, AI- and ML-driven companies, and foundational tech layers that are reshaping drug discovery and development.
Why should you read this? If you’re a founder, this is a chance to get inside the mind of an early-stage VC and understand what SaaS and technology investors look for when evaluating opportunities in TechBio, and use this knowledge (or terminology) to refine your next pitch. If you’re an investor, consider this your field guide to identifying key segments that deserve a closer look.
Our working definition of TechBio: these are technology platforms that don’t just complement scientific work but are inherently tied to advancing it—geared towards delivering an impactful end product. This spans everything from diagnostics to carbon capture. For this post we are focusing on the arena we think is best placed for disruptive growth: drug discovery and development.
How is the market evolving and which themes do we see?
To quote Patrick Möller, CIO of Bayer Pharma: „My personal bet is that AI will transform productivity for everyone in the pharma value chain by 2026.“ The conversation has moved beyond isolated success stories; it’s about rethinking how the entire industry works, from drug discovery to delivery. We’ve seen a surge of large language models (LLMs) tailored for biology, including AlphaFold cracking protein structures, Meta’s ESMFold, and BioGPT making waves in specialized research. The era of biology’s digitization is here, reinforced by the cloud’s power to virtualize samples and catalog findings like never before. The result is a massive explosion of data, boosted by the continuous advancements in DNA sequencing and analytics.
But despite the explosion of data — biopharma companies today collect seven times more investigational drug data than two decades ago — drug approval timelines have not gotten any shorter, nor have hit rates improved. The ecosystem is buzzing with different perspectives, approaches, and strategies. The value of public data? Hotly debated. Open-source models? Divisive. What is the right approach to collaboration and knowledge sharing? Still up for discussion. Whether building a structured data foundation or diving into the mess of unstructured data enhanced by LLMs: everyone is still trying to crack the code. Some, such as pharma giant Merck, start with a clear problem and build outward. Others have started by setting up AI taskforces that can end up feeling like solutions in search of a problem. And when it comes to building AI stacks, most companies are preferring to develop this critical part of the company in-house, as seen with AstraZeneca’s open-source model adoption and Qiagen’s data-to-knowledge graph pipeline.
Major Challenges
When evaluating the potential success of new TechBio companies, it’s worth considering not just the technology but also the characteristics of the market dynamics in which these companies are building. Large pharma organizations face the usual challenges — outdated infrastructure, entrenched processes, and the cultural shifts needed to embrace digital tech. Add to this the internal tug-of-war between commercial teams prioritizing boosting sales, research teams racing to complete trials, and healthcare practitioners and payors focusing on minimizing costs, and you will find different interests and goals inside of one organization.
When it comes to AI and ML, the hurdles are even higher. The data burden is real, especially with personalized medicine. Genomic data is coming from massive projects like UK Biobank, Genomics England, and All Of Us, each housed on its own clunky, proprietary platform. For small, scrappy teams, moving models from one dataset to another while ensuring reproducibility and maintaining security is a mammoth task.
And then there’s the complex question of trust. In AI, it’s not enough to say that it works—people want to know how it works. The proof is not in the pudding here; stakeholders need to understand what is in the recipe. Historically, drug research has always been a mix of science and mystery, from Fleming’s chance discovery of penicillin to the opaque leaps of combinatorial chemistry. Breakthroughs often relied on trial, error, and intuition. Today, AI revives that skepticism, with data and models operating beyond human intuition. It’s not just about results but ensuring stakeholders can trace the logic to something they trust.
Our TechBio Market Map
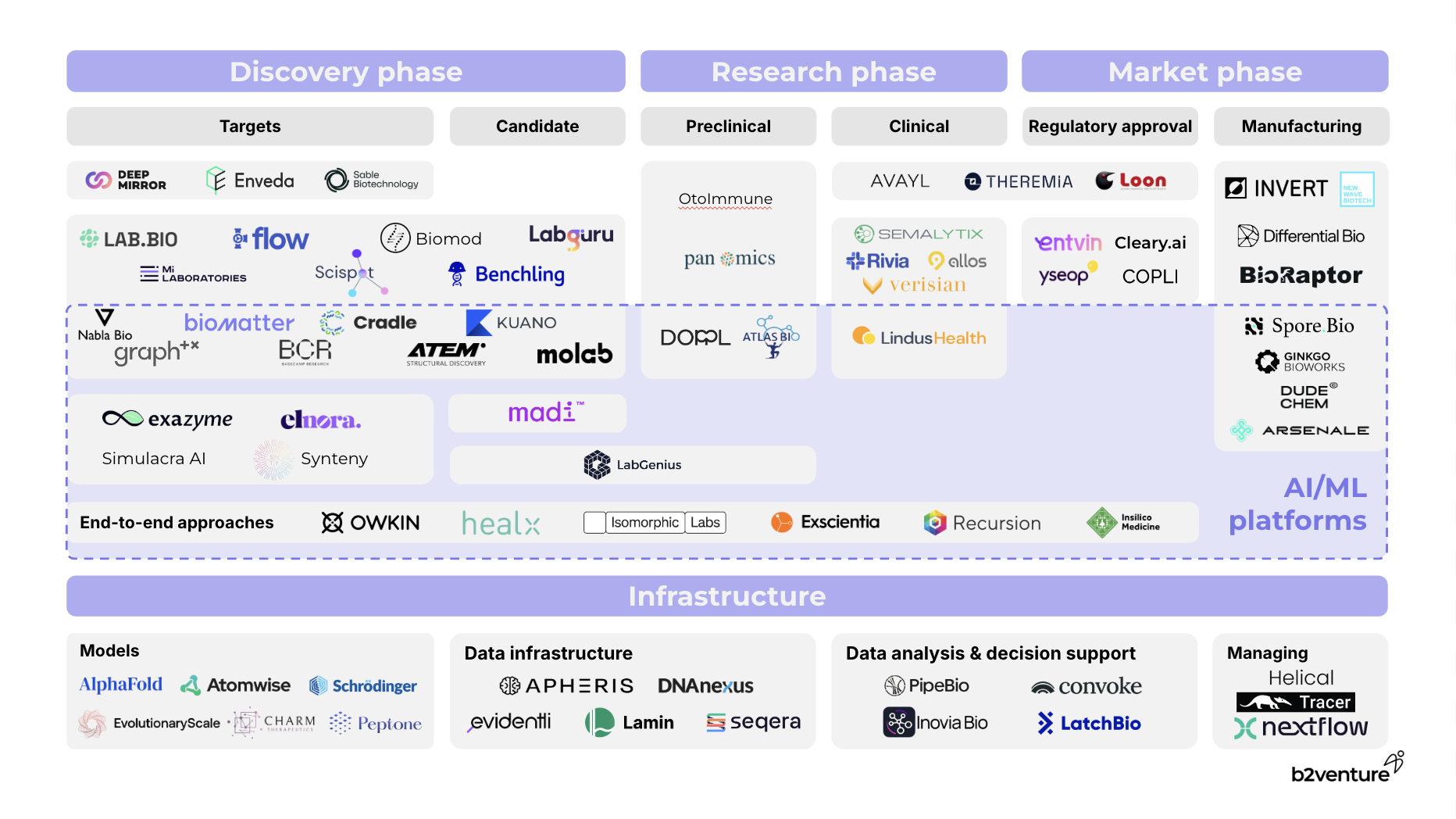
From a technology perspective, there are two major waves shaping the TechBio stack: Like the Modern Data Stack in tech (whose evolution Matt Turck has extensively explored here), the data infrastructure in life sciences is evolving and far from consolidated, echoing the early 2010s tech landscape where fragmented data solutions gradually coalesced into unified systems. Life sciences now face the challenge of integrating vast, multi-modal datasets — from genomic records to clinical trials — amidst legacy platforms, before any AI can sit on top of it. As a senior executive at Merck puts it, focusing on public data and robust infrastructure could allow the whole industry to “stand on the shoulders of giants.”
The second wave is the ML/AI innovation cycle, supercharged by the rise of LLMs and generative AI, like AlphaFold and Peptone, pushing predictive models to new heights. Compared to general tech, the relationship between data and AI in life sciences is even more deeply symbiotic — tight feedback loops are essential to validate hypotheses, guide better-targeted experiments, and optimize the selection of promising candidates, ultimately speeding up the path from discovery to development.
We find that the best way to think of the TechBio market map is as an assembly line — a continuum from discovery to development, and ultimately, value generation. Along this assembly line, we find three archetypes of TechBio companies:
- Applications (the top layer): SaaS-based platforms that provide outward-facing tools, catering to researchers and pharma companies to streamline operations of the assembly line.
- ML & AI platforms (the middle): This layer embodies the most comprehensive approach, where proprietary technology integrates seamlessly with wet lab operations, either in-house or through strategic partnerships. These platforms enable tight data feedback loops that accelerate learning cycles, bridging tech development and drug discovery. Some companies focus on specific parts of the assembly line (for now), like graphTX integrating advanced machine learning to optimize gene and cell therapy processes. Others are starting to develop an end-to-end stack, like Owkin leveraging AI models to identify novel drug targets and predict outcomes, and pipelines from first-generation TechBio companies such as Recursion that have progressed to clinical trials.
- Infrastructure (the foundation): These are the building blocks, essential for both pharma giants and startups striving for product quality. Companies like Benchling, Seqera, and Lamin Labs play pivotal roles in mining vast datasets and supporting seamless data replatforming and analysis. We’ve recently backed a team in this space - stay tuned for the announcement shortly.
Though each layer is crucial to propelling life sciences into its next era, we have a particular interest in the infrastructure layer and look forward to seeing more companies evolving in this part of the stack.
TechBio Business Models
The intersection of biotech and AI/ML technology brings a unique tension into business models, balancing two inherently conflicting worlds. BioTech traditionally centers around proprietary IP and asset development, tying value to a specific therapeutic with limited application. The binary risk of whether these assets work, tied to a long timeline, does not match the typical software VC fund profile tailored to platforms which gain traction over time. Meanwhile, technology-centric startups focus on market problems and scalable solutions, allowing for early-stage pivots—a crucial trait for growth.
AI is moving these two worlds closer together by accelerating R&D and promising faster asset development (and exits) as well as new, more scalable business strategies.
There’s a delicate decision to be made for a start-up between licensing their platform (aligning with the VC-friendly, scalable SaaS revenue model) and keeping the platform to itself to develop their own assets, which comes with significant capital needs but higher potential returns. Timing is key: early outlicensing can provide revenue and validate a company’s platform, while transitioning to in-house asset development can capture greater upside as the platform matures. For insights on platform validation and key KPIs, Pablo Lubroth’s TechBio KPIs guide is a must-read.
Which themes are we closely tracking for winners based in Europe?
While the US has deep VC pockets and aggressive M&A strategies to drive rapid late-stage development, Europe’s scientific talent is unmatched, with a network of world-renowned academic and research institutions fueling its strength in early-stage R&D. The European pharma landscape thrives on collaboration between public research institutions and private enterprises (which even US giants such as Eli Lilly are tapping into), creating a fertile environment for innovation. Success cases like Novo Nordisk show that it’s possible to capitalize on Europe’s collaborative spirit to become leaders in diabetes care and innovative treatments.
European startups excel in forming strategic partnerships with larger pharma players, as illustrated by Owkin, which collaborates with major companies to harness AI for precision medicine. The landscape is seeing an uptick in technologies spun out into commercial applications; in 2021, European TechBio startups raised $8.5bn, signaling that TechBio is starting to command a bigger slice of venture funding. Companies like LabGenius exemplify Europe’s capacity to build and scale impactful startups.
We are particularly interested in teams solving pivotal challenges with software-driven approaches:
- Trust: Driven by challenges outlined above around data scarcity and transparency how a ML/AI platform works, the European emphasis on scientific rigor and deep validation makes it fertile ground for startups that enable data transparency and the trustworthiness of ML/AI platforms.
- Data infrastructure: Europe’s collaborative ecosystem reveals the need to move beyond mere data collection (e.g., UK Biobank) and transform data into scientifically actionable insights. The key is quality over quantity—not just more models but cleaner, validated data that integrates experimental insights. Experimental data consistently outperforms silicon-generated data, and models thrive on exposure to failure (negative data) to sharpen their predictive power. Maintaining clean data, monitoring for drift, and ensuring reproducibility are essential.
- Lab automation: Given the tighter data feedback loops needed, this area is ripe for disruption, and we’ve not yet seen a larger wave of companies addressing this challenge.
If you’re a founder building in this space or an investor ready to back the next wave of TechBio innovation, let’s connect!
The Author

Marisa Krummrich
Investment Manager
Marisa is Investment Manager in the b2venture Fund team and focuses on horizontal AI, AI tooling, and vertical enterprise applications that utilize AI as the key enabler.
Team